2275 results for "earn" across all locations
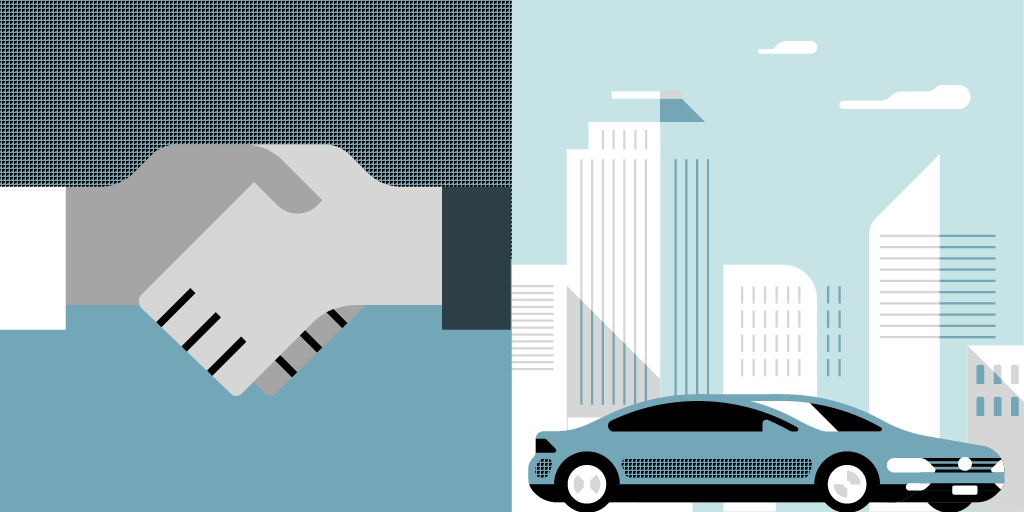
UberPITCH – Celebrating Entrepreneurship Across Europe
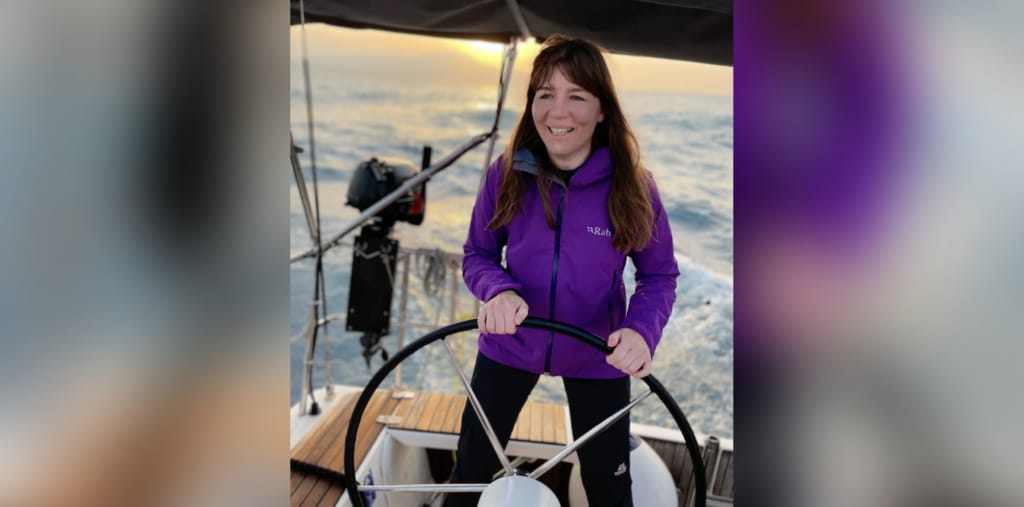
From Math to Machine Learning: Anastasia’s Tech Journey to Uber
Sr. Software Engineer Anastasia Tugaenko explains how she is engineering a new career path at Uber after attending European Women in Tech last year.
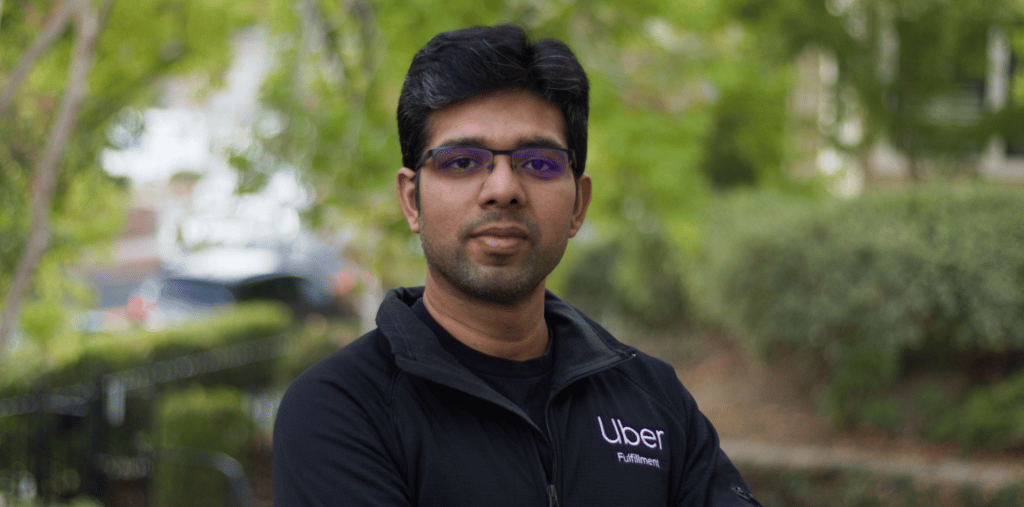
What Madan’s learned in 8 years as part of Uber engineering
Madan Thangavelu is a Director of Marketplace Engineering leading teams that power the logistics behind orders, fares, and product selection.
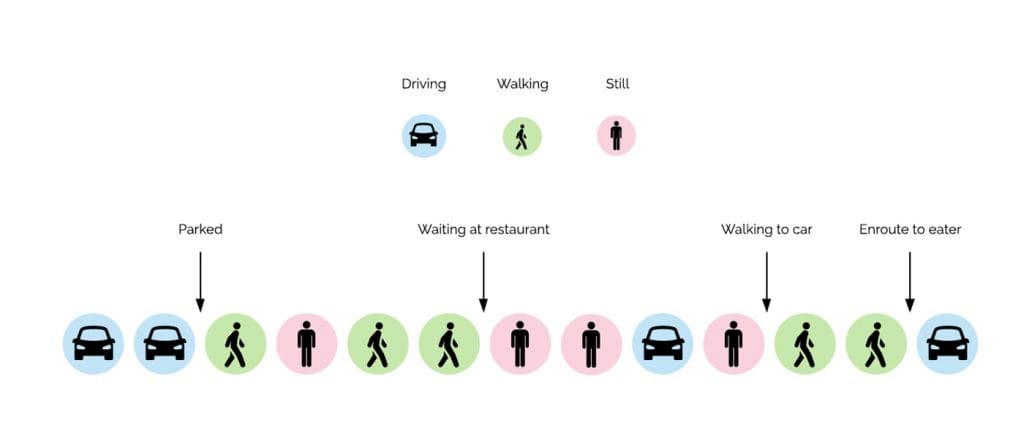
How Trip Inferences and Machine Learning Optimize Delivery Times on Uber Eats
Using GPS and sensor data from Android phones, Uber engineers develop a state model for trips taken by Uber Eats delivery-partners, helping to optimize trip timing for delivery-partners and eaters alike.
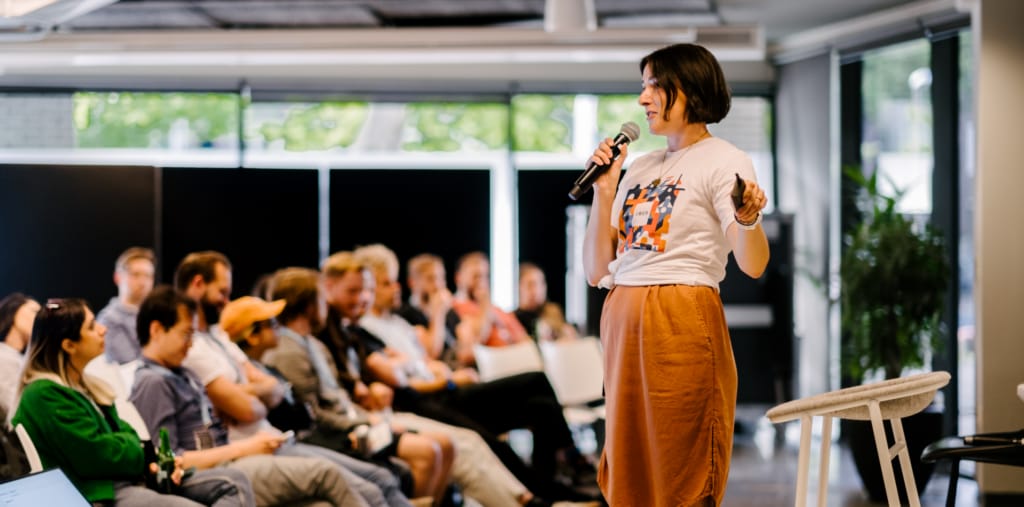
Uber design night: coming together to learn, engage, and inspire
Our latest Design Night hosted in Amsterdam spotlighted accessibility, featuring Erica Ellis and local industry leaders.
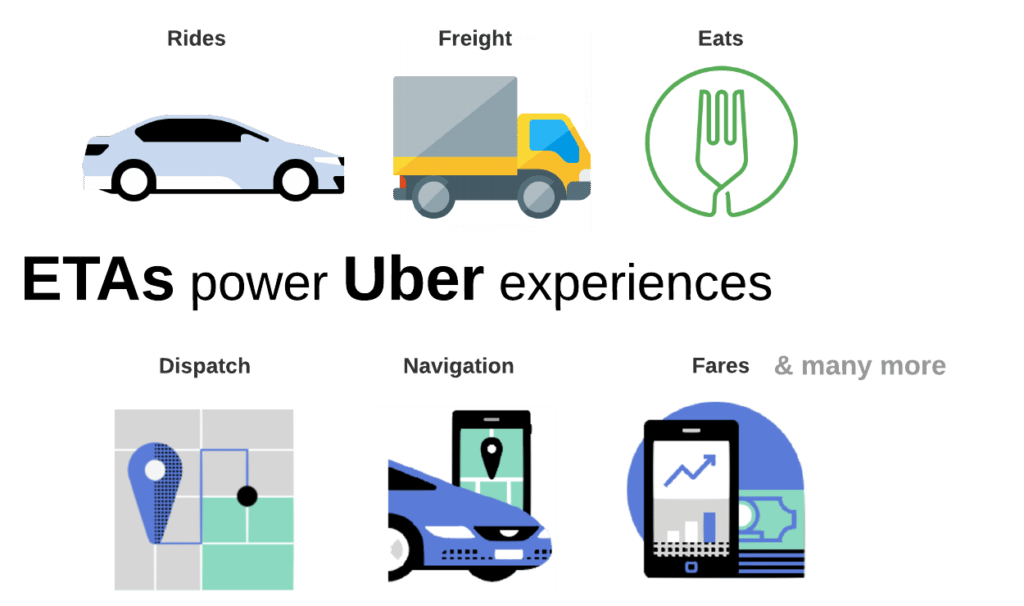
DeepETA: How Uber Predicts Arrival Times Using Deep Learning
End-to-end Learning of Multi-sensor 3D Tracking by Detection
D. Frossard, R. Urtasun
In this paper we propose a novel approach to tracking by detection that can exploit both cameras as well as LIDAR data to produce very accurate 3D trajectories. Towards this goal, we formulate the problem as a linear program that can be solved exactly, and learn convolutional networks for detection as well as matching in an end-to-end manner. […] [PDF]
International Conference on Robotics and Automation (ICRA), 2018
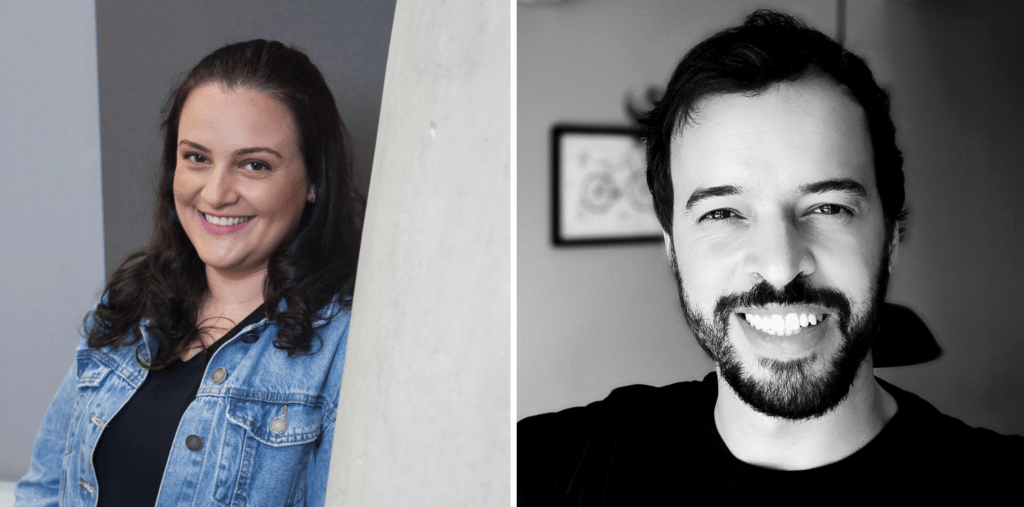
Empowering Safe Rides: An Engineering-Product Collaboration in LatAm
Learn about how Celeste, from Uber’s Product team, and Chris, from Uber’s engineering team, partnered to launch a groundbreaking new safety feature for passengers.
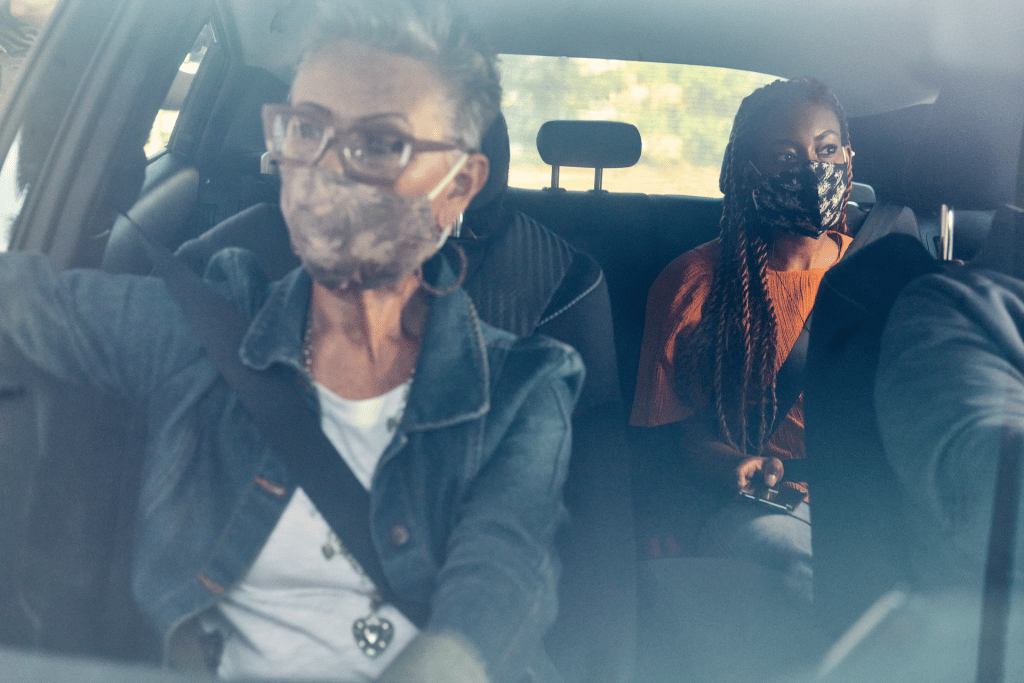
Black Girl Ventures uses Uber for Business to support its team
Black Girl Ventures is on a mission to lower the barrier to capital for entrepreneurs identifying as Black and Brown women. Learn how the company uses Uber for Business to support its employees and the founders in its network.
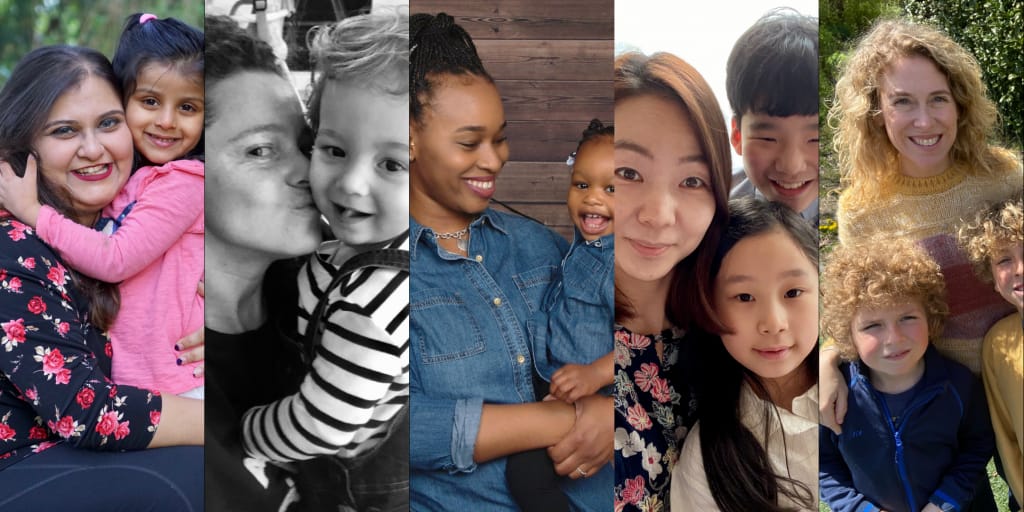
5 moms share how they balance parenting and their career
This Mother’s Day, more than a year into working from home, we checked in with five moms across the world to learn how they’re balancing parenting and growing their careers here at Uber.