Three Approaches to Scaling Machine Learning with Uber Seattle Engineering
September 11, 2019 / Global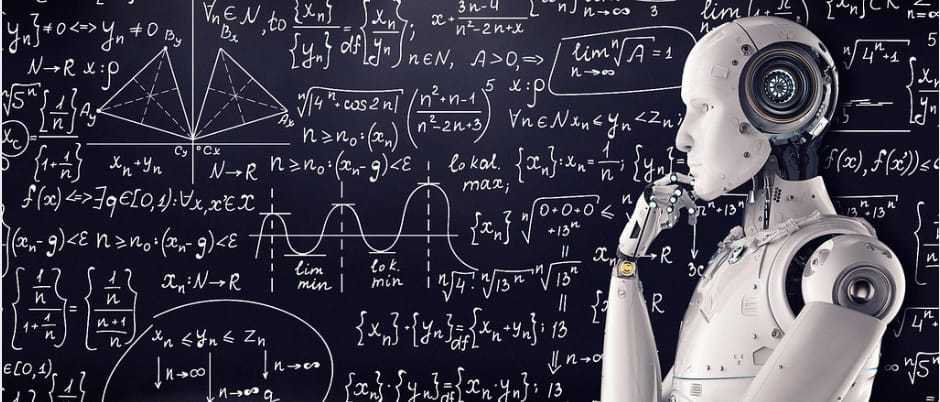
Uber’s services require real-world coordination between a wide range of customers, including driver-partners, riders, restaurants, and eaters. Accurately forecasting things like rider demand and ETAs enables this coordination, which makes our services work as seamlessly as possible.
In an effort to constantly optimize our operations, serve our customers, and train our systems to perform better and better, we leverage machine learning (ML). In addition, we make many of our ML tools open source, sharing them with the community to advance the state of the art.
In this spirit, members of our Seattle Engineering team shared their work at an April 2019 meetup on ML and AI at Uber. Below, we highlight three different approaches Uber Seattle Engineering is currently working on to improve our ML ecosystem and that of the tech community at large.
Horovod: Distributed Deep Learning on Apache Spark
During his talk, senior software engineer Travis Addair, from the ML Platform team, describes the power of deep learning and explains how Horovod, an open source deep learning framework built at Uber, helps facilitate this important function, especially when used with Apache Spark. As a distributed training platform, Horovod allows companies to scale their ML to hundreds of machines. Horovod’s unique abstracted framework also helps infrastructure professionals and ML engineers focus on doing their best work without stepping on each other’s digital toes. Travis details how Horovod’s deep learning systems work and demonstrates why NVIDIA, Amazon, Alibaba, ORNL, and other major players are using it for their own ML platforms.
Michelangelo (MA) Learners and Transformers
Senior software engineer Mingshi Wang introduces the audience to various logical and physical workflows and models in Michelangelo, Uber’s ML-as-a-service platform. He explains how Michelangelo’s flexible learners and transformers simplify the process of ML while providing outstanding results. As with Travis’s talk on Horovod, Mingshi focuses on Michelangelo’s compatibility and synergy with Apache Spark. He also showcases Michelangelo’s intuitive interface and the insights its dashboard offers. His presentation concludes by describing Uber’s plans to make Michelangelo’s transformers, workflows, and other features open source in the near future, enabling others to leverage their advantages.
Long-Term Rider Behavior Modeling using Pyro
Data scientist Hesen Peng demonstrates how to model and predict rider behavior for Uber’s rewards programs using Pyro, an open source probabilistic programming language built by Uber. Between walking viewers through various ML-powered models, providing a live coding demonstration, highlighting Pyro’s recursive simulation capabilities, and discussing how to model with censored time-to-event data with Pyro, Hesen references some of his favorite Uber memes.
To learn more about ML at Uber, we welcome you to read our other articles on the subject (and keep an eye out for future ones).
Do you want to collaborate with us to help develop the future of ML? Consider applying for a position with Uber’s Seattle office!
Header image courtesy of www.vpnsrus.com, licensed under Creative Commons 2.0.
Posted by Lucy
Related articles
Most popular
Introducing the new Uber Eats Pro – better service, better status, better earnings
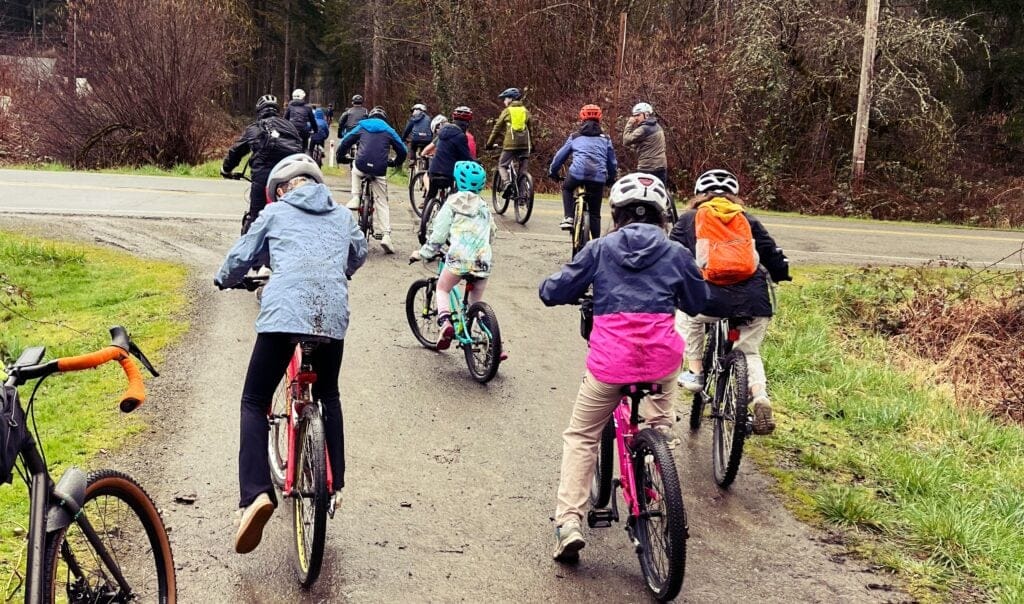