2220 results for "earn" across all locations
Graph Partition Neural Networks for Semi-Supervised Classification
R. Liao, M. Brockschmidt, D. Tarlow, A. Gaunt, R. Urtasun, R. Zemel
We present graph partition neural networks (GPNN), an extension of graph neural networks (GNNs) able to handle extremely large graphs. GPNNs alternate between locally propagating information between nodes in small subgraphs and globally propagating information between the subgraphs. […] [PDF]
Workshop @ International Conference on Machine Learning (ICLR), 2018
Magnetic Hamiltonian Monte Carlo
N. Tripuraneni, M. Rowland, Z. Ghahramani, R. Turner
Hamiltonian Monte Carlo (HMC) exploits Hamiltonian dynamics to construct efficient proposals for Markov chain Monte Carlo (MCMC). In this paper, we present a generalization of HMC which exploits textit{non-canonical} Hamiltonian dynamics. […] [PDF]
International Conference on Machine Learning (ICML), 2017
A birth-death process for feature allocation
K. Palla, D. Knowles, Z. Ghahramani
We propose a Bayesian nonparametric prior over feature allocations for sequential data, the birthdeath feature allocation process (BDFP). The BDFP models the evolution of the feature allocation of a set of N objects across a covariate (e.g. time) by creating and deleting features. […] [PDF]
International Conference on Machine Learning (ICML), 2017
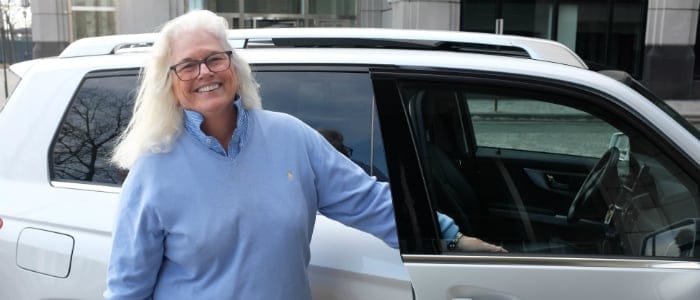
Behind the Wheel with…Maureen, NJ uberX Partner
Maureen, a New Jersey native, worked on Wall Street for 31 years until finally she decided that she needed a break. Today, you can find Maureen cruising the streets of New Jersey—from down the shore (where she lives) to Hoboken and Jersey City—driving as one of our highest-rated uberX partners. Learn more about Maureen.
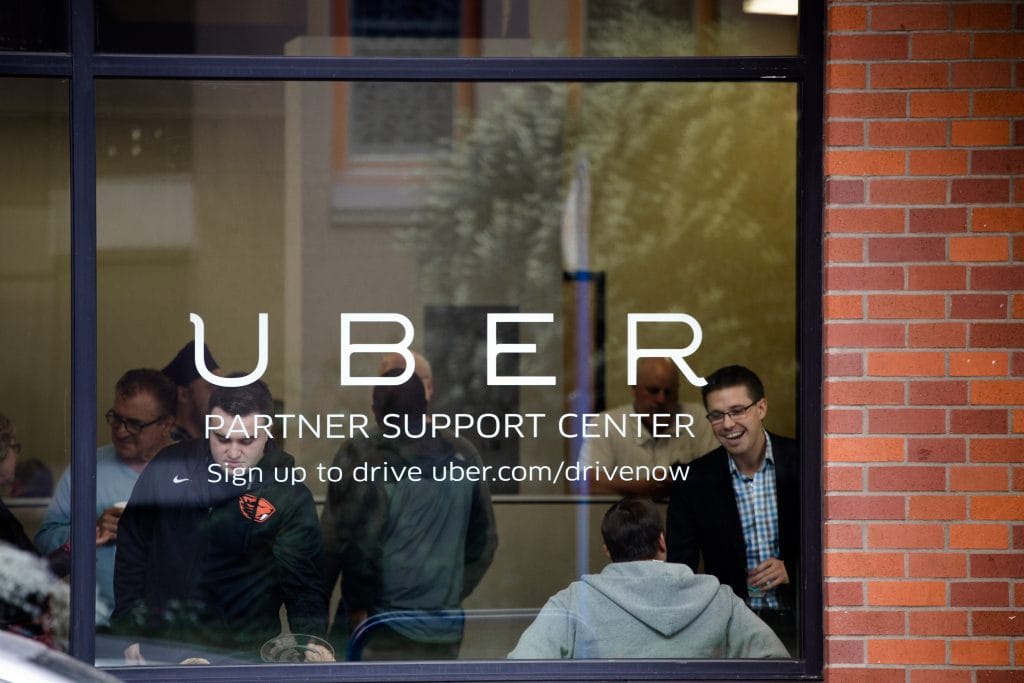
Meet your Portland Drivers
With over 5,000 partners in Portland and counting, it’s nice to take some time and get to know their stories. Through events at our Partner Support Center, volunteer opportunities and partner involvement with UberIMPACT, we’ve learned a bit more about the people who make Uber in Portland possible. Read some of their stories below:
UPSNet: A Unified Panoptic Segmentation Network
Y. Xiong, R. Liao, H. Zhao, R. Hu, M. Bai, E. Yumer, R. Urtasun
In this paper we tackle the problem of scene flow estimation in the context of self-driving. We leverage deep learning techniques as well as strong priors as in our application domain the motion of the scene can be composed by the motion of the robot and the 3D motion of the actors in the scene. […] [PDF]
Conference on Computer Vision and Pattern Recognition (CVPR), 2019
Be Your Own Prada: Fashion Synthesis With Structural Coherence
S. Zhu, R. Urtasun, S. Fidler, D. Lin, C. Loy
We present a novel and effective approach for generating new clothing on a wearer through generative adversarial learning. Given an input image of a person and a sentence describing a different outfit, our model “redresses” the person as desired, while at the same time keeping the wearer and her/his pose unchanged. […] [PDF]
International Conference on Computer Vision (ICCV), 2017
Lost Relatives of the Gumbel Trick
M. Balog, N. Tripuraneni, Z. Ghahramani, A. Weller
The Gumbel trick is a method to sample from a discrete probability distribution, or to estimate its normalizing partition function. The method relies on repeatedly applying a random perturbation to the distribution in a particular way, each time solving for the most likely configuration. […] [PDF]
International Conference on Machine Learning (ICML), 2017
HDNET: Exploiting HD Maps for 3D Object Detection
B. Yang, M. Liang, R. Urtasun
In this paper we show that High-Definition (HD) maps provide strong priors that can boost the performance and robustness of modern 3D object detectors. Towards this goal, we design a single stage detector that extracts geometric and semantic features from the HD maps. […] [PDF]
Conference on Robot Learning (CORL), 2018
Lower Prices; Increased Demand
Every city has its busy times and times when things are slow. New York City is no exception. In the summer, the city empties out as people head off on vacation. In the fall, it’s full of tourists doing their holiday shopping. And by January, once the sales have finished, things tend to quiet down again. Whatever a city’s seasonality, the down times can be tough for drivers. So today we’re cutting uberX rates by 15%. Learn more.