2217 results for "earn" across all locations
Uber Flash: Se cadastre ou indique motoristas de utilitários, caminhonetes, vans ou pequenos caminhões!
Comece a realizar viagens Uber Flash Utilitário, Van ou Carreto
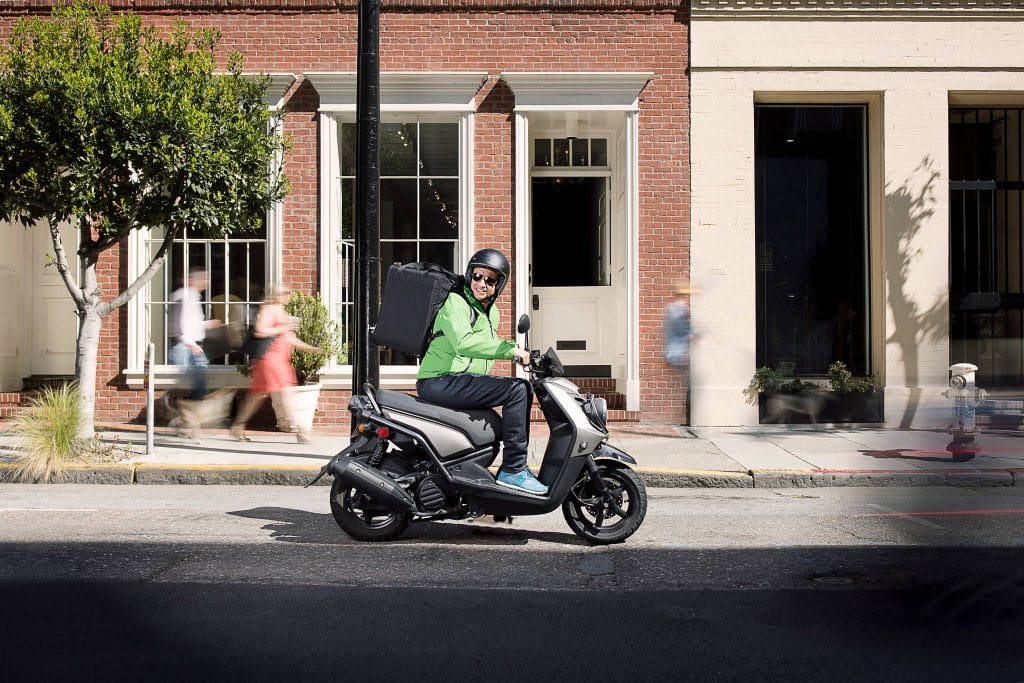
額外趟次挑戰
Uber Eats 外送合作夥伴可在夥伴端 App 內的「選單 > 機會」中查看您專屬的額外趟次挑戰獎勵活動,每位外送合作夥伴所看到的獎勵方案不盡相同。外送合作夥伴保有是否參與本獎勵活動、滿足本獎勵活動條款或完成獎勵行程趟次之決定。
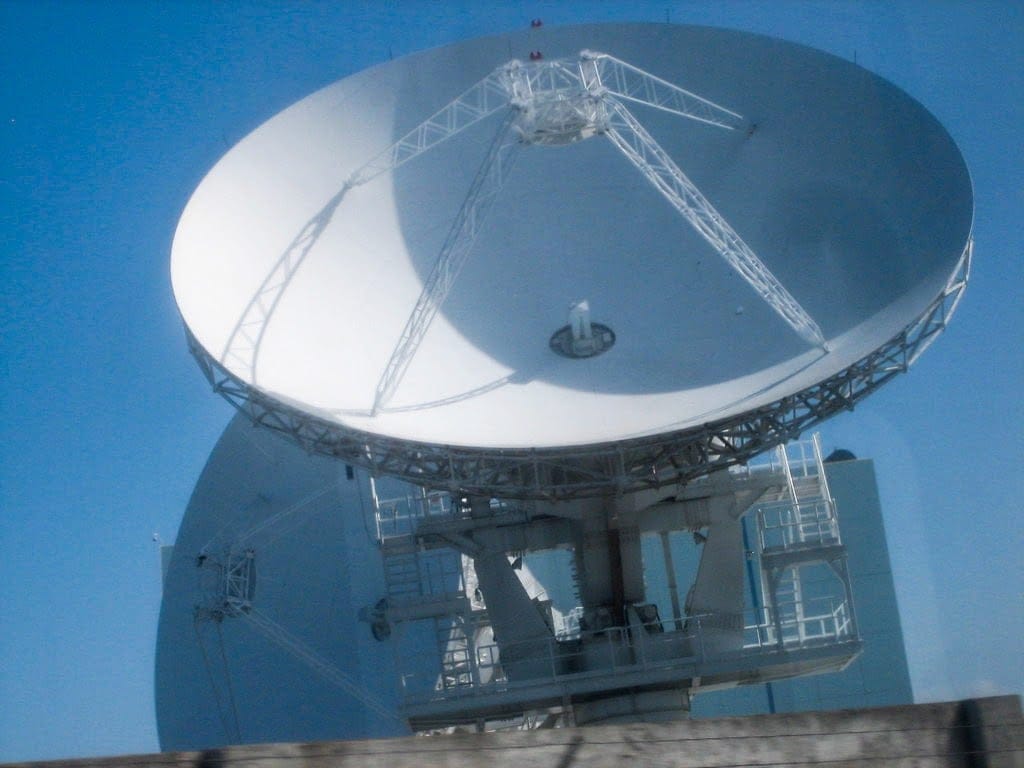
Optimal Feature Discovery: Better, Leaner Machine Learning Models Through Information Theory
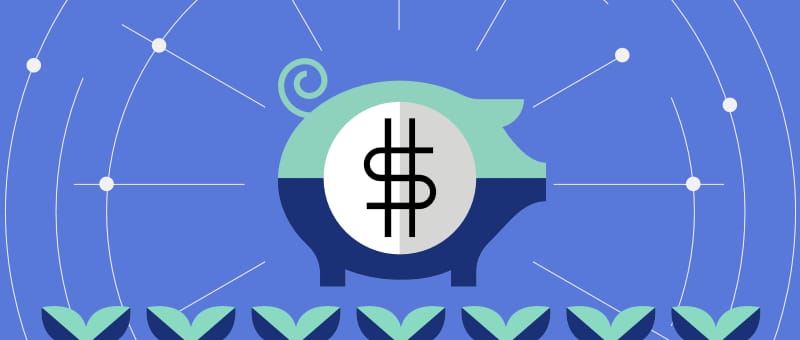
Uber x betterment: flexible options to save for the future
Let’s face it: saving — especially for the long term — is tough. Whether it’s an unexpected bill or a weekend splurge, too often the money we meant to stash away for tomorrow tends to find its way out the door today. That’s why we’re excited to partner with Betterment to offer flexible retirement accounts to Uber driver partners. Learn how to make the most with every mile…
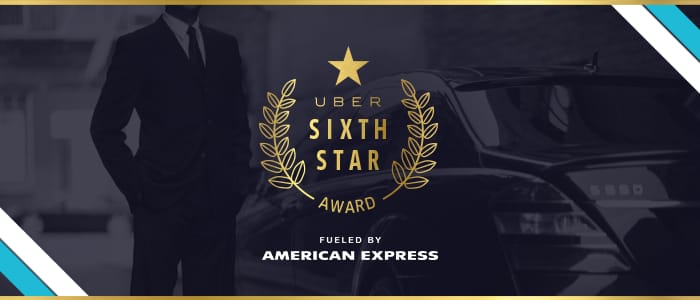
Finally, A Way To Give Your Driver A Sixth Star
Every day across the nation, drivers on the Uber platform work hard to seamlessly and efficiently move people around their cities. And sometimes, in the midst of getting you from point A to point B, a driver does something amazing.
Today, Uber and American Express are excited to honor these incredible drivers with our “Sixth Star Award” program. Hear their stories and learn more…
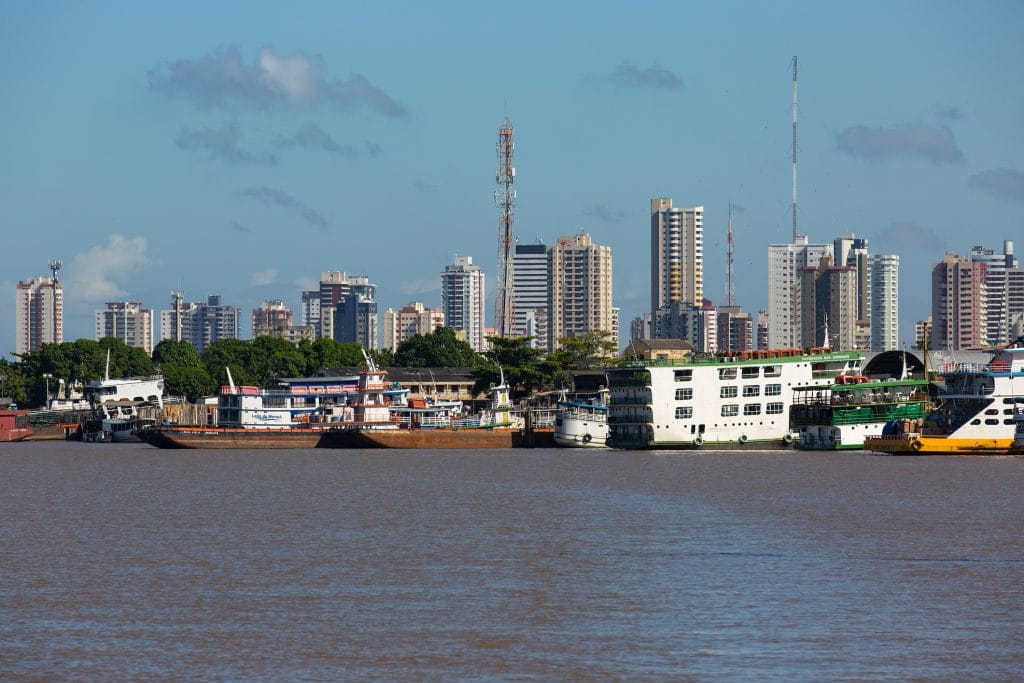
Confira o que fazer em Belém e aproveite o melhor da cidade
Confira algumas dicas sobre o que fazer em Belém. Encante-se com a capital paraense e confira as principais coisas para fazer em Belém.
Measuring the Intrinsic Dimension of Objective Landscapes
Chunyuan Li, Heerad Farkhoor, R. Liu, J. Yosinski
Many recently trained neural networks employ large numbers of parameters to achieve good performance. One may intuitively use the number of parameters required as a rough gauge of the difficulty of a problem. But how accurate are such notions? How many parameters are really needed? In this paper we attempt to answer this question by training networks not in their native parameter space, but instead in a smaller, randomly oriented subspace. […] [PDF]
International Conference on Learning Representations (ICLR), 2018
Probabilistic Meta-Representations Of Neural Networks
T. Karaletsos, P. Dayan, Z. Ghahramani
Existing Bayesian treatments of neural networks are typically characterized by weak prior and approximate posterior distributions according to which all the weights are drawn independently. Here, we consider a richer prior distribution in which units in the network are represented by latent variables, and the weights between units are drawn conditionally on the values of the collection of those variables. […] [PDF]
UAI 2018 Uncertainty In Deep Learning Workshop (UDL), 2018
Pathwise Derivatives Beyond the Reparameterization Trick
M. Jankowiak, F. Obermeyer
We observe that gradients computed via the reparameterization trick are in direct correspondence with solutions of the transport equation in the formalism of optimal transport. We use this perspective to compute (approximate) pathwise gradients for probability distributions not directly amenable to the reparameterization trick: Gamma, Beta, and Dirichlet. […] [PDF]
International Conference on Machine Learning (ICML), 2018
Deconstructing Lottery Tickets: Zeros, Signs, and the Supermask
H. Zhou, J. Lan, R. Liu, J. Yosinski
Optical Character Recognition (OCR) approaches have been widely advanced in recent years thanks to the resurgence of deep learning. The state-of-the-art models are mainly trained on the datasets consisting of the constrained scenes. Detecting and recognizing text from the real-world images remains a technical challenge. […] [PDF]
Conference on Neural Information Processing Systems (NeurIPS), 2019