Designing Schemaless, Uber Engineering’s Scalable Datastore Using MySQL
January 12, 2016 / Global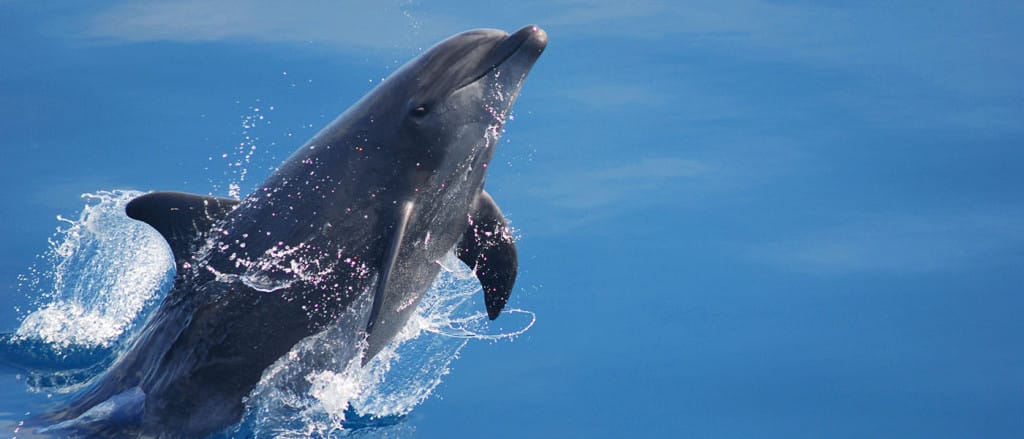
The making of Schemaless, Uber Engineering’s custom designed datastore using MySQL, which has allowed us to scale from 2014 to beyond. This is part one of a three-part series on Schemaless.
In Project Mezzanine we described how we migrated Uber’s core trips data from a single Postgres instance to Schemaless, our fault-tolerant and highly available datastore. This article further describes its architecture and the expanded role Schemaless has had in Uber’s infrastructure, and how it came to be.
Our Race for a New Database
In early 2014, we were running out of database space due to flourishing trip growth. Each new city and trip milestone pushed us to the precipice, to the point where we realized Uber’s infrastructure would fail to function by the end of the year: we simply couldn’t store enough trip data with Postgres. Our mission was to implement the next generation of database technology for Uber, a task that took many months, much of the year, and involved many engineers from our engineering offices around the world.
But first, why even build a scalable datastore when a wealth of commercial and open source alternatives already exist? We had five key requirements for our new trip data store:
- Our new solution needed to be able to linearly add capacity by adding more servers, a property our Postgres setup lacked. Adding servers should both increase the available disk storage and decrease the system response times.
- We needed write availability. We had previously implemented a simple buffer mechanism with Redis, so if the write to Postgres failed we could retry later since the trip had been stored in Redis in the interim. But while in Redis, the trip could not be read from Postgres and we lost functionality like billing. Annoying, but at least we did not lose the trip! As time passed, Uber grew, so our Redis-based solution did not scale. Schemaless had to support a similar mechanism as Redis, but favoring write availability over read-your-write semantics.
- We needed a way of notifying downstream dependencies. In the current system, we had processed many trip components simultaneously (e.g., billing, analytics, etc.). This was an error-prone process: if any step failed, we had to retry all over again even if some components were successful. This does not scale, so we wanted to break out these steps into isolated steps, initiated by data changes. We did have an asynchronous trip event system, but it was based on Kafka 0.7. We were not able to run it lossless, so we would welcome a new system that had something similar, but could run lossless.
- We needed secondary indexes. As we were moving away from Postgres, the new storage system had to support Postgres indexes, which meant secondary indexes to search for trips in the same manner.
- We needed operation trust in the system, as it contains mission-critical trip data. If we get paged at 3 am when the datastore is not answering queries and takes down the business, would we have the operational knowledge to quickly fix it?
In light of the above, we analyzed the benefits and potential limitations of some alternative commonly-used systems, such as Cassandra, Riak, and MongoDB, etc. For purposes of illustration, a chart is provided below showing different combinations of capabilities of different system options:
Linearly scales | Write availability | Notification | Indexes | Ops Trust | |
Option 1 | ✓ | ✓ | ✗ | (✓) | ✗ |
Option 2 | ✓ | ✓ | ✗ | (✓) | (✓) |
Option 3 | ✓ | ✗ | ✗ | (✓) | ✗ |
While all three systems are able to scale linearly by adding new nodes online, only a couple systems can also receive writes during failover. None of the solutions have a built-in way of notifying downstream dependencies of changes, so we would need to implement that at the application level. They all have indexes, but if you’re going to index many different values, the queries become slow, as they use scatter-gather to query all nodes. Lastly some systems that we had experience using were single clusters, were not serving user-facing online traffic, and had various operational issues in connection with our services.
Finally, our decision ultimately came down to operational trust in the system we’d use, as it contains mission-critical trip data. Alternative solutions may be able to run reliably in theory, but whether we would have the operational knowledge to immediately execute their fullest capabilities factored in greatly to our decision to develop our own solution to our Uber use case. This is not only dependent on the technology we use, but also the experience with it that we had on the team.
We should note that since we surveyed the options more than two years ago and found none applicable for the trip storage use case, we have now adopted both Cassandra and Riak with success in other areas of our infrastructure, and we use them in production to serve many millions of users at scale.
In Schemaless We Trust
As none of the above options fulfilled our requirements under the timeframe we were given, we decided to build our own system that is as simple as possible to operate, while applying the scaling lessons learned from others. The design is inspired by Friendfeed, and the focus on the operational side inspired by Pinterest.
We ended up building a key-value store which allows you to save any JSON data without strict schema validation, in a schemaless fashion (hence the name). It has append-only sharded MySQL with buffered writes to support failing MySQL masters and a publish-subscribe feature for data change notification which we call triggers. Lastly, Schemaless supports global indexes over the data. Below, we discuss an overview of the data model and some key features, including the anatomy of a trip at Uber, with more in depth examples reserved for a followup article.
The Schemaless Data Model
Schemaless is an append-only sparse three dimensional persistent hash map, very similar to Google’s Bigtable. The smallest data entity in Schemaless is called a cell and is immutable; once written, it cannot be overwritten or deleted. The cell is a JSON blob referenced by a row key, a column name, and a reference key called ref key. The row key is a UUID, while the column name is a string and the reference key is an integer.
You can think of the row key as a primary key in a relational database, and the column name as a column. However, in Schemaless there is no predefined or enforced schema and rows do not need to share column names; in fact, the column names are completely defined by the application. The ref key is used to version the cells for a given row key and column. So if a cell needs to be updated, you would write a new cell with a higher ref key (the latest cell is the one with the highest ref key). The ref key is also useable as entries in a list, but is typically used for versioning. The application decides which scheme to employ here.
Applications typically group related data into the same column, and then all cells in each column have roughly the same application-side schema. This grouping is a great way to bundle data that changes together, and it allows the application to rapidly change the schema without downtime on the database side. The example below elaborates more on this.
Example: Trip Storage in Schemaless
Before we dive into how we model a trip in Schemaless, let’s look at the anatomy of a trip at Uber. Trip data is generated at different points in time, from pickup drop-off to billing, and these various pieces of info arrive asynchronously as the people involved in the trip give their feedback, or background processes execute. The diagram below is a simplified flow of when the different parts of an Uber trip occur:
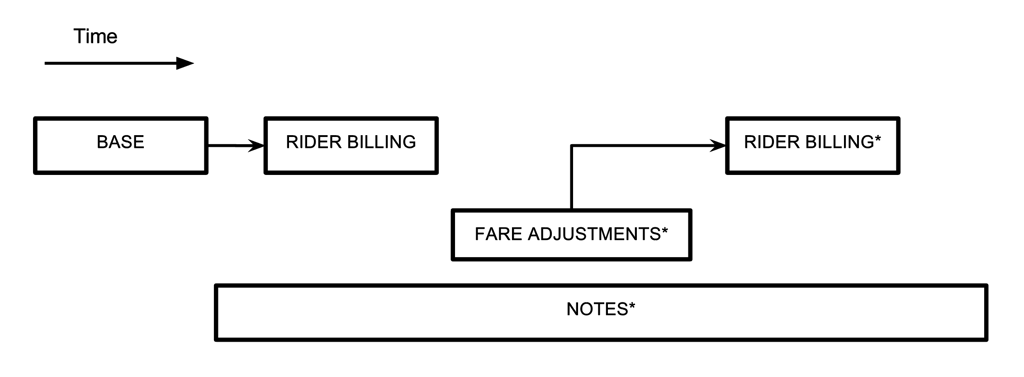
A trip is driven by a partner, taken by a rider, and has a timestamp for its beginning and end. This info constitutes the base trip, and from this we calculate the cost of the trip (the fare), which is what the rider is billed. After the trip ends, we might have to adjust the fare, where we either credit or debit the rider. We might also add notes to it, given feedback from the rider or driver (shown with asterisks in the diagram above). Or, we might have to attempt to bill multiple credit cards, in case the first is expired or denied. Trip flow at Uber is a data driven process. As data becomes available or is added, then certain set of processes will execute on the trip. Some of this info, such as a rider or driver rating (considered part of the notes in the above diagram), could arrive days after the trip finished.
So, how do we map the above trip model into Schemaless?
The Trip Data Model
Using italics to denote UUIDs and UPPERCASE to denote column names, the table below shows the data model for a simplified version of our trip store. We have two trips (UUIDs trip_uuid1 and trip_uuid2) and four columns (BASE, STATUS, NOTES, and FARE ADJUSTMENT). A cell is represented by a box, with a number and a JSON blob (abbreviated with {…}). The boxes are overlaid to represent the versioning (i.e., the differing ref keys).
trip_uuid1 has three cells: one in the BASE column, two in the STATUS column and none in the FARE ADJUSTMENTs column. trip_uuid2 has two cells in the BASE column, one in the NOTES column, and likewise none in the FARE ADJUSTMENTS column. For Schemaless, the columns are not different; so the semantics of the columns are defined by the application, which in this case is the service Mezzanine.
In Mezzanine, the BASE column cells contain basic trip info, such as the driver’s UUID and the trip’s time of day. The STATUS column contains the trip’s current payment status, where we insert a cell for each attempt to bill the trip. (An attempt could fail if the credit card does not have sufficient funds or has expired.). The NOTES column contains a cell if there are any notes associated with the trip by the driver or left by an Uber DOps (Driver Operations employee). Lastly the FARE ADJUSTMENTs column contains cells if the trip fare has been adjusted.
We use this column split to avoid data races and minimize the amount of data that needs to be written on updates. The BASE column is written when a trip is finished, and thus typically only once. The STATUS column is written when we attempt to bill the trip which happens after the data in the BASE column is written and can happen several times if the billing fails. The NOTES column can similarly be written multiple times at some point after the BASE is written, but it is completely separate from the STATUS column writes. Similarly, the FARE ADJUSTMENTS column is only written if the trip fare is changed, for example due to an inefficient route.
Schemaless Triggers
A key feature of Schemaless is triggers, the ability to get notified about changes to a Schemaless instance. Since the cells are immutable and new versions are appended, each cell also represents a change or a version, allowing the values in an instance to be viewed as a log of changes. For a given instance, it is possible to listen on these changes and trigger functions based on them, very much like an event bus system such as Kafka.
Schemaless triggers make Schemaless a perfect source-of-truth data store because, besides random access to the data, downstream dependencies can use the trigger feature to monitor and trigger any application-specific code (a similar system is LinkedIn’s DataBus), hence decoupling data creation and its processing.
Among other use cases, Uber uses Schemaless triggers to bill a trip when its BASE column is written to the Mezzanine instance. Given the example above, when the BASE column for trip_uuid1 is written, our billing service that triggers on the BASE column picks this cell up and will try to bill the trip by charging the credit card. The result of charging the credit card, whether it is a success or a failure, is written back to Mezzanine in the STATUS column. This way the billing service is decoupled from the creation of the trip, and Schemaless acts as an asynchronous event bus.
Indexes for Easy Access
Finally, Schemaless supports indexes defined over the fields in the JSON blobs. An index is queried via these predefined fields to find cells that match the query parameters. Querying these indexes is efficient, because the index query only need go to a single shard to find the set of cells to return. In fact, the queries can further be optimized, as Schemaless allows the cell data to be denormalized directly into the index. Having the denormalized data in the index means that an index query only need to consult one shard for both querying and retrieving the information. In fact, we typically recommend Schemaless users to denormalize data that they might think they need into the indexes in case they need to query any information besides retrieving a cell directly via the row key. In a sense, we thereby trade storage for fast query lookup.
As an example for Mezzanine, we have a secondary index defined allowing us to find a given driver’s trips. We have denormalized the trip creation time and the city where the trip was taken. This makes it possible to find all the trips for a driver in a city within a given time range. Below we give the definition of the driver_partner_index in YAML format, that is part of the trips datastore and defined over the BASE column (the example is annotated with comments using the standard #).
Using this index, we can find trips for a given driver_partner_uuid filtered by either city_uuid and/or trip_created_at. In this example we only use fields from the BASE column, but Schemaless supports denormalizing data from multiple columns, which would amount to multiple entries in the above column_def list.
As mentioned Schemaless has efficient indexes, achieved by sharding the indexes based on a sharding field. Therefore the only requirement for an index is that one of the fields in the index is designated as a shard field (in the above example that would be driver_partner_uuid, as it is the first given). The shard field determines which shard the index entry should be written to or retrieved from. The reason is that we need to supply the shard field when querying an index. That means on query time we only need to consult one shard for retrieving the index entries. One thing to note about the sharding field is that it should have a good distribution. UUIDs are best, city ids are suboptimal and status fields (enums) are detrimental to the storage.
For other than the shard field, Schemaless supports equality, non-equality and range queries for filtering, and supports selecting only a subset of the fields in the index and retrieving specific or all columns for the row key that the index entries points to. Currently, the shard field must be immutable, so Schemaless always only need to talk with one shard. But, we’re exploring how to make it mutable without too big of a performance overhead.
The indexes are eventually consistent; whenever we write a cell we also update the index entries, but it does not happen in the same transaction. The cells and the index entries typically do not belong to the same shard. So if we were to offer consistent indexes, we would need to introduce 2PC in the writes, which would incur significant overhead. With eventually consistent indexes we avoid the overhead, but Schemaless users may see stale data in the indexes. Most of the time the lag is well below 20ms between cell changes and the corresponding index changes.
Summary
We’ve given an overview of the data model, triggers and indexes, all of which are key features which define Schemaless, the main components of our trip storage engine. In future posts, we’ll look at a few other features of Schemaless to illustrate how it’s been a welcome companion to the services in Uber’s infrastructure: more on architecture, the use of MySQL as a storage node, and how we make triggering fault-tolerant on the client-side.
Photo Credits for Header: “anim1069” by NOAA Photo Library licensed under CC-BY 2.0. Image cropped for header dimensions and color corrected.
Header Explanation: Since Schemaless is built using MySQL, we introduce the series using a dolphin striking a similar pose but with opposite orientation to the MySQL logo.
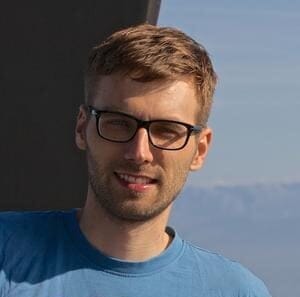
Jakob Holdgaard Thomsen
Jakob Holdgaard Thomsen is a Principal Engineer at Uber, working out of the Aarhus office, helping to make Uber's systems more performant and more reliable.
Posted by Jakob Holdgaard Thomsen
Related articles
Most popular
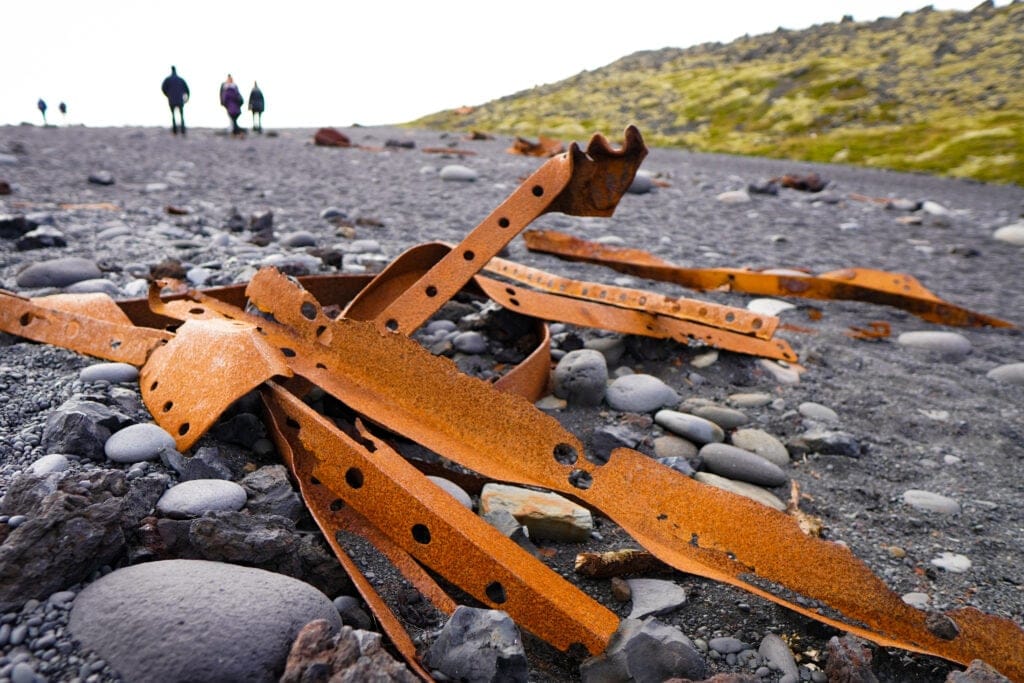
Enhanced Agentic-RAG: What If Chatbots Could Deliver Near-Human Precision?
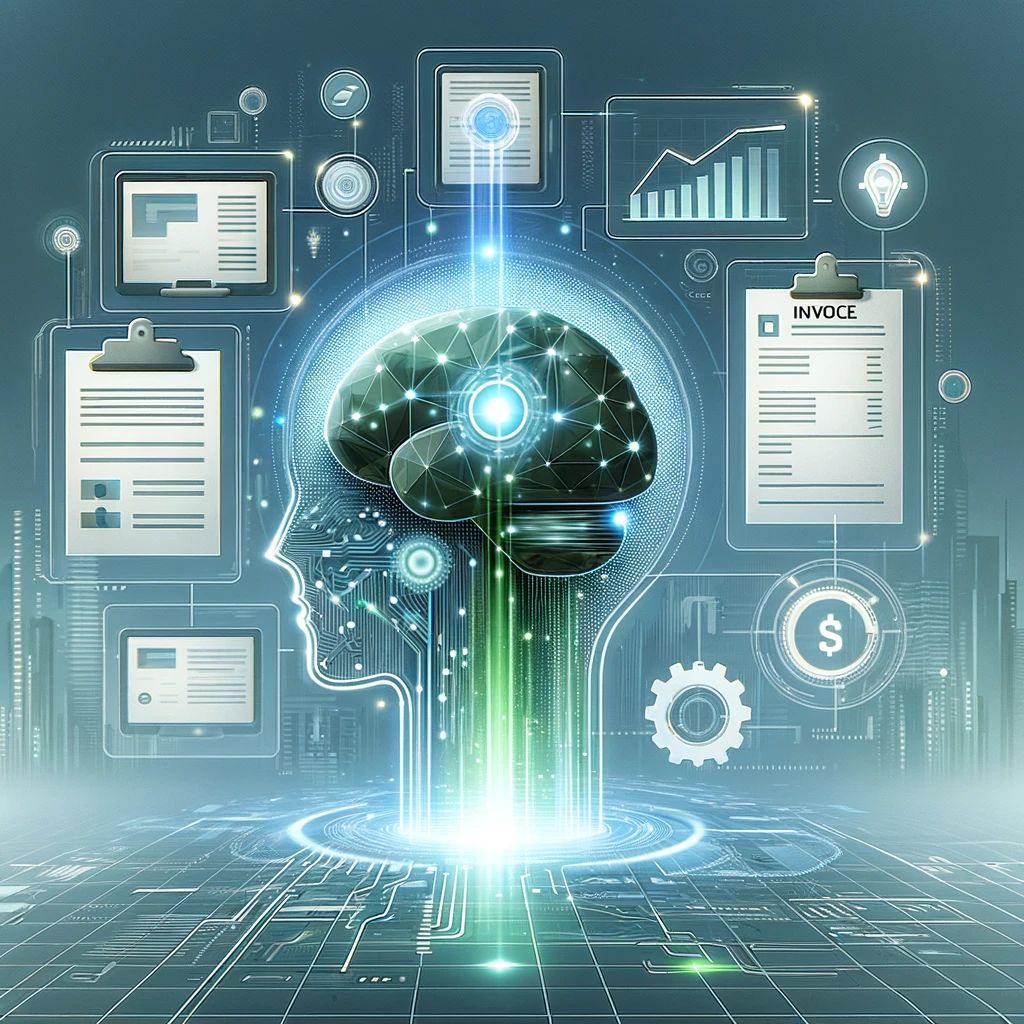
Advancing Invoice Document Processing at Uber using GenAI
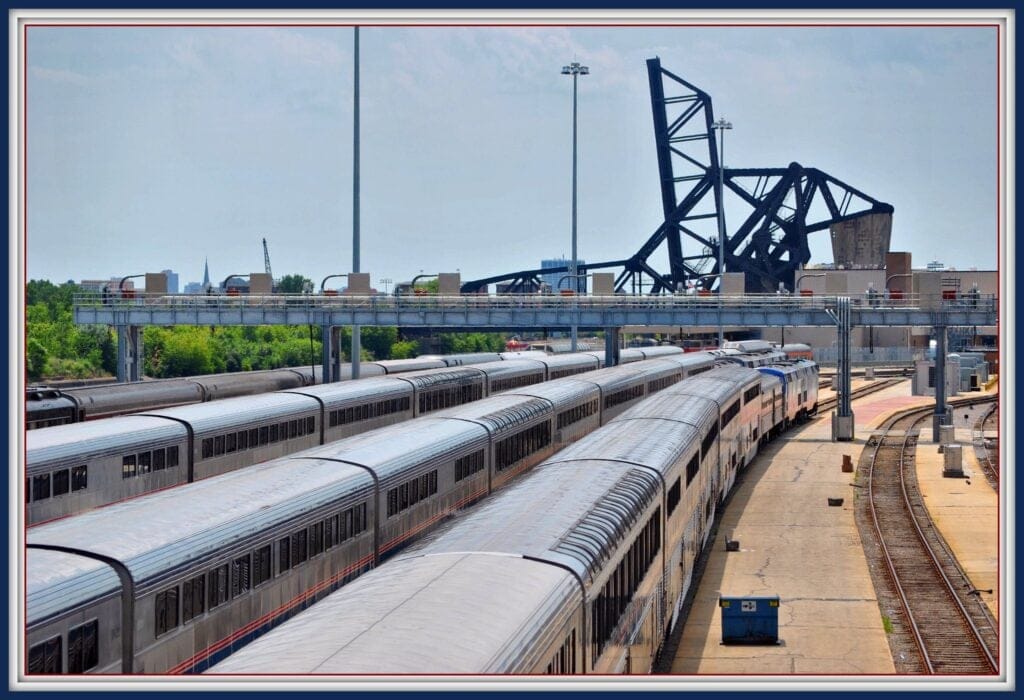
From Archival to Access: Config-Driven Data Pipelines
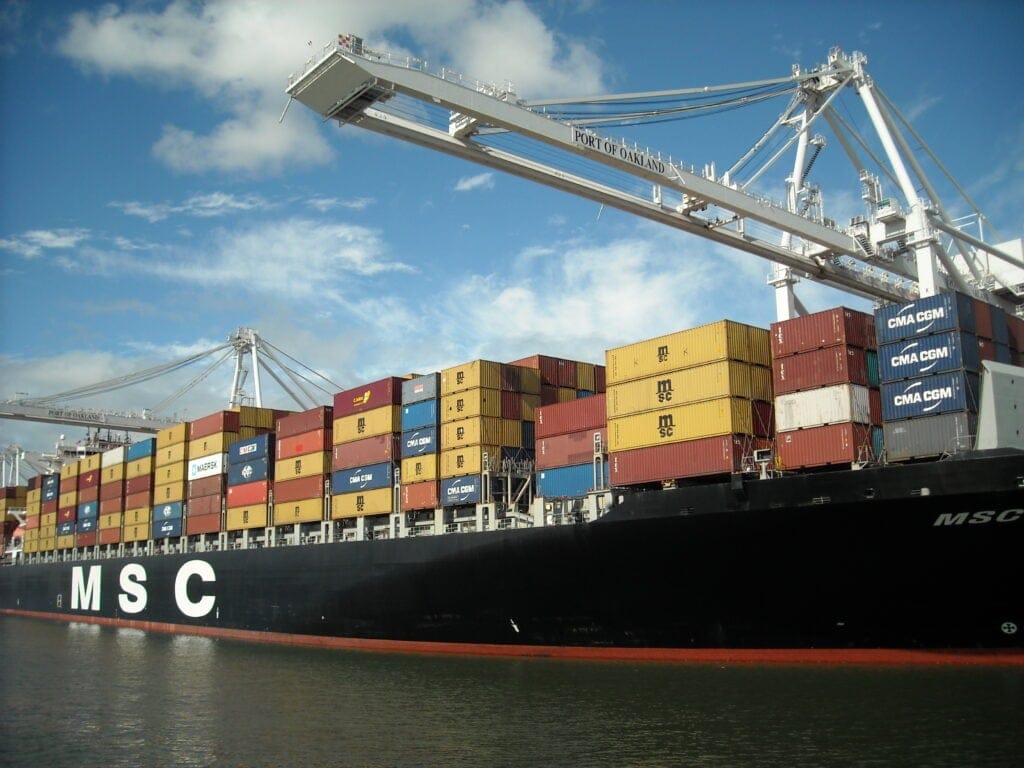