Introducing the Plato Research Dialogue System: A Flexible Conversational AI Platform
July 16, 2019 / Global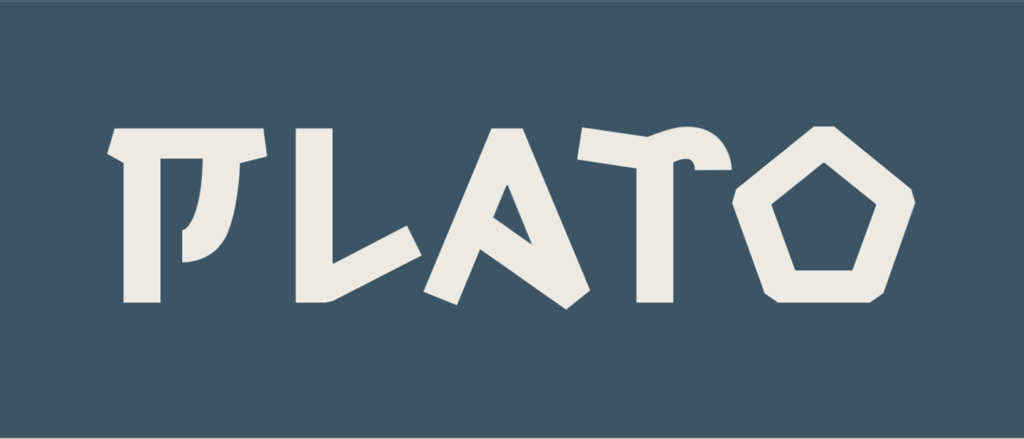
Intelligent conversational agents have evolved significantly over the past few decades, from keyword-spotting interactive voice response (IVR) systems to the cross-platform intelligent personal assistants that are becoming an integral part of daily life.
Along with this growth comes the need for intuitive, flexible, and comprehensive research and development platforms that can act as open testbeds to help evaluate new algorithms, quickly prototype, and reliably deploy conversational agents.
At Uber AI, we developed the Plato Research Dialogue System, a platform for building, training, and deploying conversational AI agents that allows us to conduct state of the art research in conversational AI and quickly create prototypes and demonstration systems, as well as facilitate conversational data collection. We designed Plato for both users with a limited background in conversational AI and seasoned researchers in the field by providing a clean and understandable design, integrating with existing deep learning and Bayesian optimization frameworks (for tuning the models), and reducing the need to write code.
There have been numerous efforts to develop such platforms for general research or specific use cases, including Olympus, PyDial, ParlAI, the Virtual Human Toolkit, Rasa, DeepPavlov, ConvLab, among others. When assessing whether or not to leverage these tools, we found that many entail that users be familiar with the platform-specific source code, focus on specific use cases and neither flexibly nor scalably support others, and require licenses to use.
Plato was designed to address these needs and can be used to create, train, and evaluate conversational AI agents for a variety of use cases. It supports interactions through speech, text, or structured information (in other words, dialogue acts), and each conversational agent can interact with human users, other conversational agents (in a multi-agent setting), or data. Perhaps most significantly, Plato can wrap around existing pre-trained models for every component of a conversational agent, and each component can be trained online (during the interaction) or offline (from data).
How does the Plato Research Dialogue System work?
Conceptually, a conversational agent needs to go through various steps in order to process information it receives as input (e.g., “What’s the weather like today?”) and produce an appropriate output (“Windy but not too cold.”). The primary steps, which correspond to the main components of a standard architecture (see Figure 1), are:
- Speech recognition (transcribe speech to text)
- Language understanding (extract meaning from that text)
- State tracking (aggregate information about what has been said and done so far)
- API call (search a database, query an API, etc.)
- Dialogue policy (generate abstract meaning of agent’s response)
- Language generation (convert abstract meaning into text)
- Speech synthesis (convert text into speech)
Plato has been designed to be as modular and flexible as possible; it supports traditional as well as custom conversational AI architectures, and importantly, enables multi-party interactions where multiple agents, potentially with different roles, can interact with each other, train concurrently, and solve distributed problems.
Figures 1 and 2, below, depict example Plato conversational agent architectures when interacting with human users and with simulated users. Interacting with simulated users is a common practice used in the research community to jump-start learning (i.e., learn some basic behaviors before interacting with humans). Each individual component can be trained online or offline using any machine learning library (for instance, Ludwig, TensorFlow, or PyTorch) as Plato is a universal framework. Ludwig, Uber’s open source deep learning toolbox, makes for a good choice, as it does not require writing code and is fully compatible with Plato.
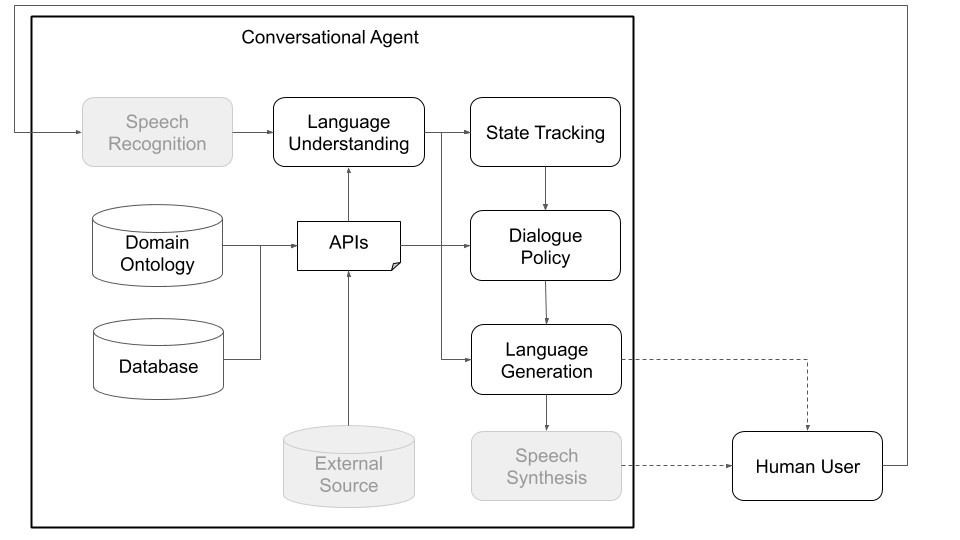
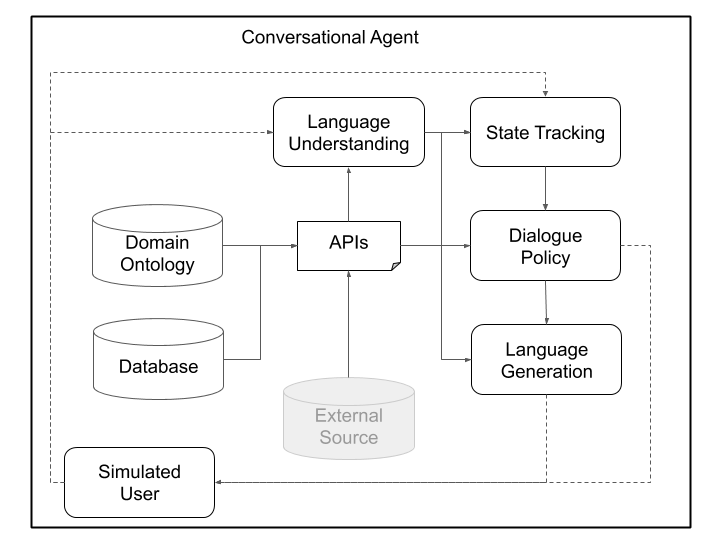
In addition to single-agent interactions, Plato supports multi-agent conversations where multiple Plato agents can interact with and learn from each other. Specifically, Plato will spawn the conversational agents, make sure that inputs and outputs (what each agent hears and says) are passed to each agent appropriately, and keep track of the conversation.
This set-up can facilitate research in multi-agent learning, where agents need to learn how to generate language in order to perform a task, as well as research in sub-fields of multi-party interactions (dialogue state tracking, turn taking, etc.). The dialogue principles define what each agent can understand (an ontology of entities or meanings; for example: price, location, preferences, cuisine types, etc.) and what it can do (ask for more information, provide some information, call an API, etc.). The agents can communicate over speech, text, or structured information (dialogue acts) and each agent has its own configuration. Figure 3, below, depicts this architecture, outlining the communication between two agents and the various components:
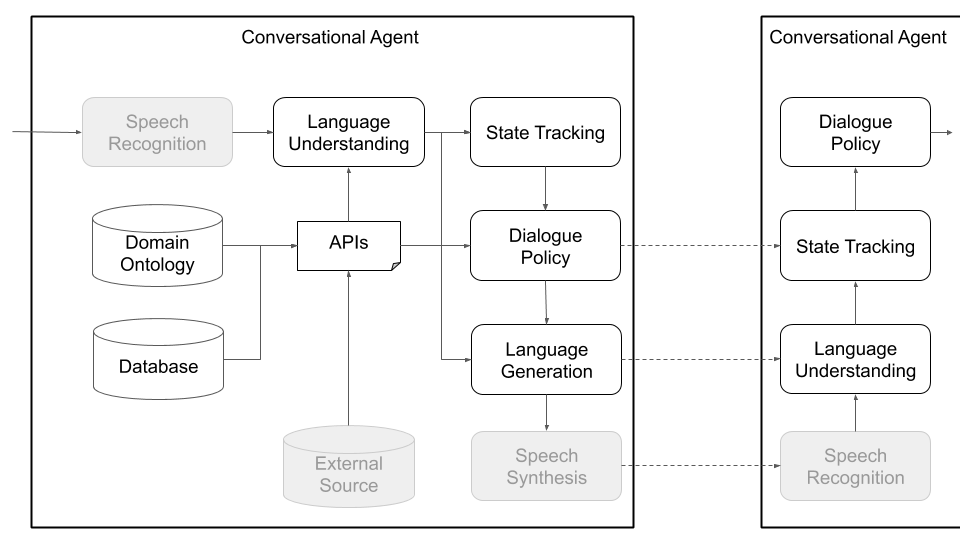
Finally, Plato supports custom architectures (e.g., splitting NLU into multiple independent components) and jointly-trained components (e.g., text-to-dialogue state, text-to-text, or any other combination) via the generic agent architecture shown in Figure 4, below:
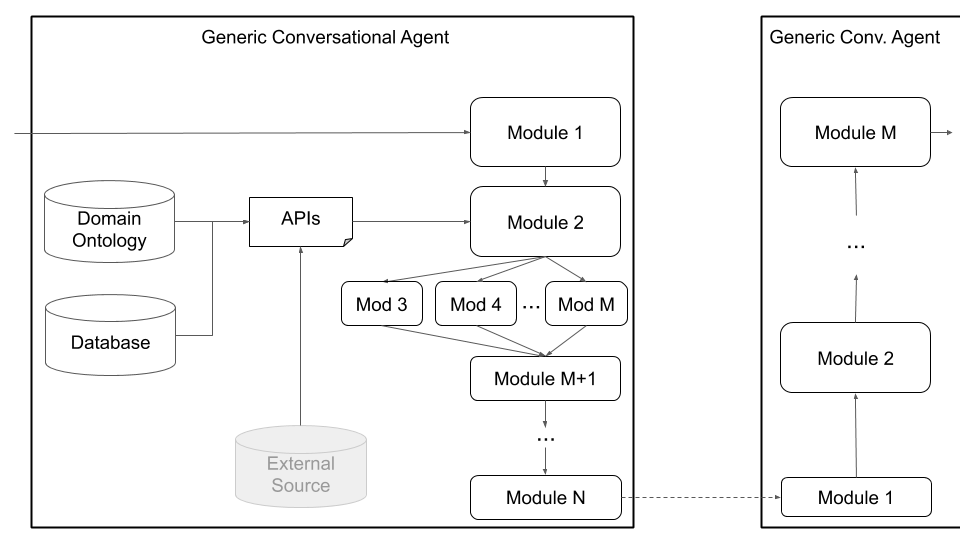
This mode moves away from the standard conversational agent architecture and supports any kind of architecture (e.g., with joint components, text-to-text or speech-to-speech components, or any other set-up) and allows loading existing or pre-trained models into Plato.
Users can define their own architecture and/or plug their own components into Plato by simply providing a Python class name and package path to that module, as well as the model’s initialization arguments. All the user needs do is list the modules in the order they should be executed and Plato takes care of the rest, including wrapping the input/output, chaining the modules, and handling the dialogues. Plato supports both serial and parallel execution of modules.
Plato also provides support for Bayesian optimization of conversational AI architectures or individual module parameters through Bayesian Optimization of Combinatorial Structures (BOCS).
Conversational agents with Plato
No actual installation is necessary in this version of Plato (v. 0.1), as it allows users to modify parts of the code or extend existing use cases for greater flexibility. However, Plato does depend on some external libraries and these need to be installed. Follow the two steps below to complete the process:
Note: The Plato Research Dialogue System has been developed with Python 3.
- Clone the repository:
git clone
git@github.com:uber-research/plato-research-dialogue-system.git
- Install the requirements:
For MacOS:
brew install portaudio
pip install -r requirements.txt
For Ubuntu/Debian:
sudo apt-get install python3-pyaudio
pip install -r requirements.tx
For Windows: