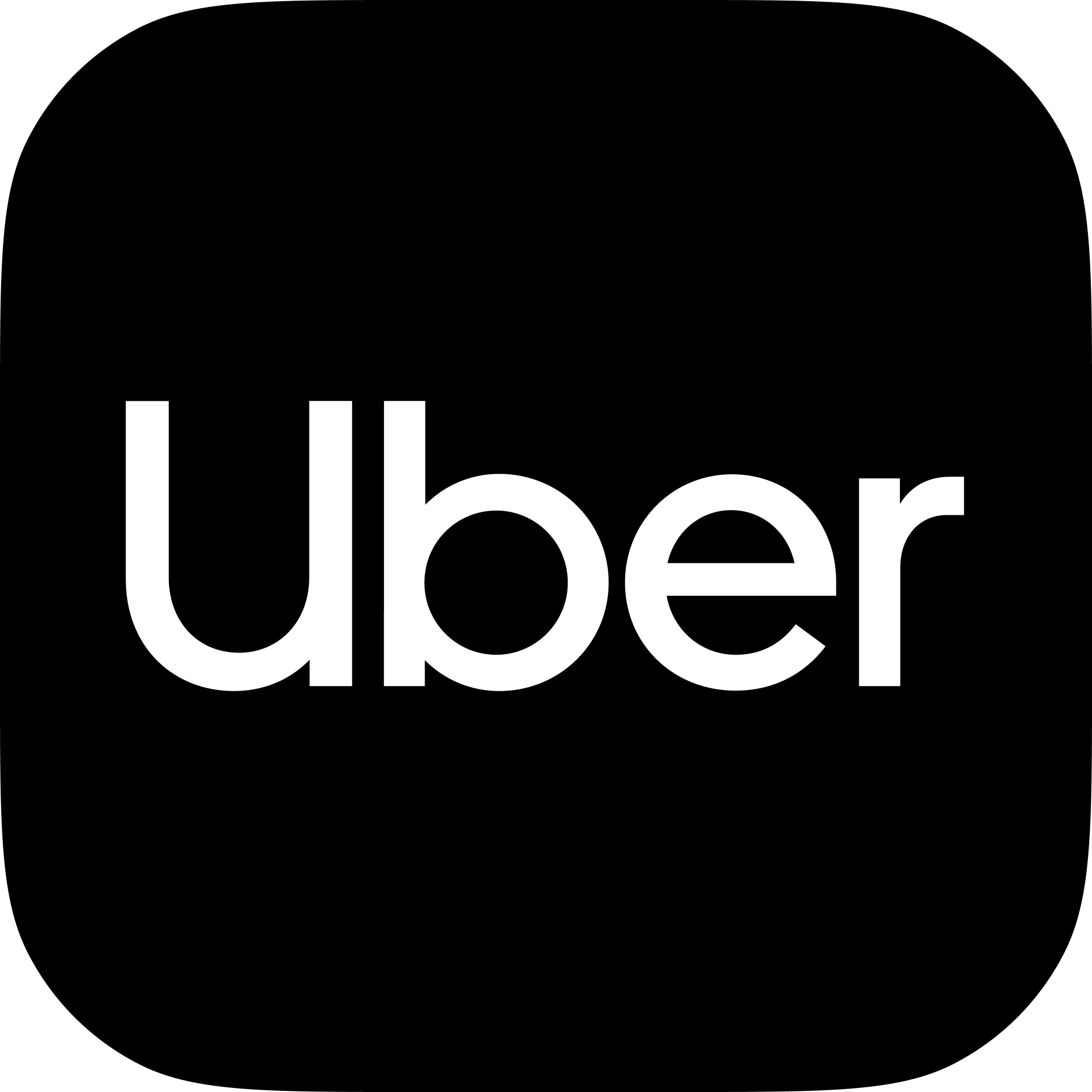
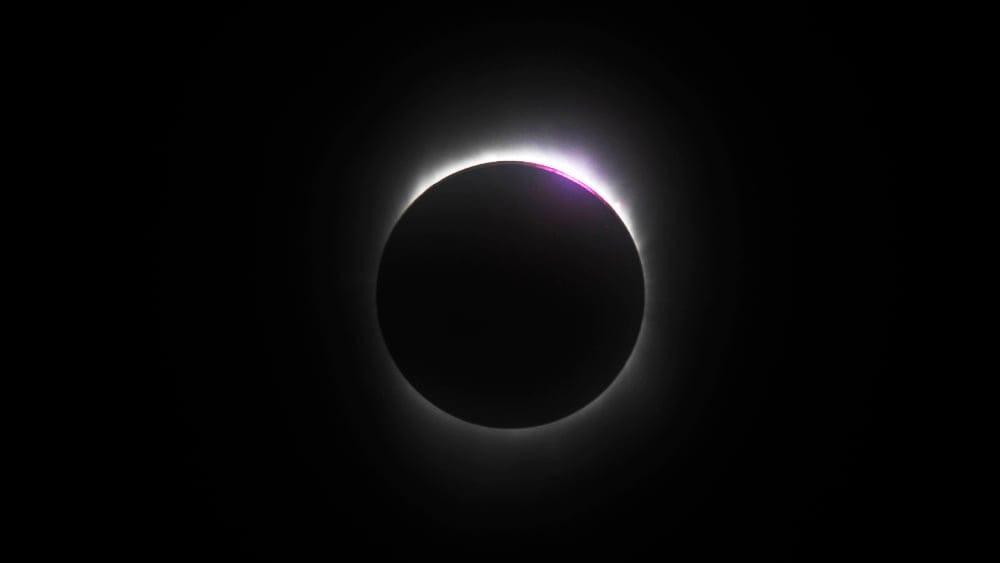
This article is the first in a series dedicated to explaining how Uber leverages forecasting to build better products and services. In recent years, machine learning, deep learning, and probabilistic programming have shown great promise in generating accurate forecasts. In addition to standard statistical algorithms, Uber builds forecasting solutions using these three techniques. Below, we discuss the critical components of forecasting we use, popular methodologies, backtesting, and prediction intervals.
Forecasting is ubiquitous. In addition to strategic forecasts, such as those predicting revenue, production, and spending, organizations across industries need accurate short-term, tactical forecasts, such as the amount of goods to be ordered and number of employees needed, to keep pace with their growth. Not surprisingly, Uber leverages forecasting for several use cases, including:
- Marketplace forecasting: A critical element of our platform, marketplace forecasting enables us to predict user supply and demand in a spatio-temporal fine granular fashion to direct driver-partners to high demand areas before they arise, thereby increasing their trip count and earnings. Spatio-temporal forecasts are still an open research area.

- Hardware capacity planning: Hardware under-provisioning may lead to outages that can erode user trust, but over-provisioning can be very costly. Forecasting can help find the sweet spot: not too many and not too few.
- Marketing: It is critical to understand the marginal effectiveness of different media channels while controlling for trends, seasonality, and other dynamics (e.g., competition or pricing). We leverage advanced forecasting methodologies to help us build more robust estimates and to enable us to make data-driven marketing decisions at scale.
What makes forecasting (at Uber) challenging?
The Uber platform operates in the real, physical world, with its many actors of diverse behavior and interests, physical constraints, and unpredictability. Physical constraints, like geographic distance and road throughput move forecasting from the temporal to spatio-temporal domains.
Although a relatively young company (eight years and counting), Uber’s hypergrowth has made it particularly critical that our forecasting models keep pace with the speed and scale of our operations.
Figure 2, below, offers an example of Uber trips data in a city over 14 months. You can notice a lot of variability, but also a positive trend and weekly seasonality (e.g., December often has more peak dates because of the sheer number of major holidays scattered throughout the month).

If we zoom in (Figure 3, below) and switch to hourly data for the month of July 2017, you will notice both daily and weekly (7*24) seasonality. You may notice that weekends tend to be more busy.

Forecasting methodologies need to be able to model such complex patterns.
Prominent forecasting approaches
Apart from qualitative methods, quantitative forecasting approaches can be grouped as follows: model-based or causal classical, statistical methods, and machine learning approaches.
Model-based forecasting is the strongest choice when the underlying mechanism, or physics, of the problem is known, and as such it is the right choice in many scientific and engineering situations at Uber. It is also the usual approach in econometrics, with a broad range of models following different theories.
When the underlying mechanisms are not known or are too complicated, e.g., the stock market, or not fully known, e.g., retail sales, it is usually better to apply a simple statistical model. Popular classical methods that belong to this category include ARIMA (autoregressive integrated moving average), exponential smoothing methods, such as Holt-Winters, and the Theta method, which is less widely used, but performs very well. In fact, the Theta method won the M3 Forecasting Competition, and we also have found it to work well on Uber’s time series (moreover, it is computationally cheap).
In recent years, machine learning approaches, including quantile regression forests (QRF), the cousins of the well-known random forest, have become part of the forecaster’s toolkit. Recurrent neural networks (RNNs) have also been shown to be very useful if sufficient data, especially exogenous regressors, are available. Typically, these machine learning models are of a black-box type and are used when interpretability is not a requirement. Below, we offer a high level overview of popular classical and machine learning forecasting methods:
Classical & Statistical | Machine Learning |
|
|
Interestingly, one winning entry to the M4 Forecasting Competition was a hybrid model that included both hand-coded smoothing formulas inspired by a well known the Holt-Winters method and a stack of dilated long short-term memory units (LSTMs).
Actually, classical and ML methods are not that different from each other, but distinguished by whether the models are more simple and interpretable or more complex and flexible. In practice. classical statistical algorithms tend to be much quicker and easier-to-use.
At Uber, choosing the right forecasting method for a given use case is a function of many factors, including how much historical data is available, if exogenous variables (e.g., weather, concerts, etc.) play a big role, and the business needs (for example, does the model need to be interpretable?). The bottom line, however, is that we cannot know for sure which approach will result in the best performance and so it becomes necessary to compare model performance across multiple approaches.
Comparing forecasting methods
It is important to carry out chronological testing since time series ordering matters. Experimenters cannot cut out a piece in the middle, and train on data before and after this portion. Instead, they need to train on a set of data that is older than the test data.

With this in mind, there are two major approaches, outlined in Figure 4, above: the sliding window approach and the expanding window approach. In the sliding window approach, one uses a fixed size window, shown here in black, for training. Subsequently, the method is tested against the data shown in orange.
On the other hand, the expanding window approach uses more and more training data, while keeping the testing window size fixed. The latter approach is particularly useful if there is a limited amount of data to work with.
It is also possible, and often best, to marry the two methods: start with the expanding window method and, when the window grows sufficiently large, switch to the sliding window method.
Many evaluation metrics have been proposed in this space, including absolute errors and percentage errors, which have a few drawbacks. One particularly useful approach is to compare model performance against the naive forecast. In the case of a non-seasonal series, a naive forecast is when the last value is assumed to be equal to the next value. For a periodic time series, the forecast estimate is equal to the previous seasonal value (e.g., for an hourly time series with weekly periodicity the naive forecast assumes the next value is at the current hour one week ago).
To make choosing the right forecasting method easier for our teams, the Forecasting Platform team at Uber built a parallel, language-extensible backtesting framework called Omphalos to provide rapid iterations and comparisons of forecasting methodologies.
The importance of uncertainty estimation
Determining the best forecasting method for a given use case is only one half of the equation. We also need to estimate prediction intervals. The prediction intervals are upper and lower forecast values that the actual value is expected to fall between with some (usually high) probability, e.g. 0.9. We highlight how prediction intervals work in Figure 5, below:

In Figure 5, the point forecasts shown in purple are exactly the same. However, the prediction intervals in the the left chart are considerably narrower than in the right chart. The difference in prediction intervals results in two very different forecasts, especially in the context of capacity planning: the second forecast calls for much higher capacity reserves to allow for the possibility of a large increase in demand.
Prediction intervals are just as important as the point forecast itself and should always be included in your forecasts. Prediction intervals are typically a function of how much data we have, how much variation is in this data, how far out we are forecasting, and which forecasting approach is used.
Moving forward
Forecasting is critical for building better products, improving user experiences, and ensuring the future success of our global business. It goes without saying that there are endless forecasting challenges to tackle on our Data Science teams. In future articles, we will delve into the technical details of these challenges and the solutions we’ve built to solve them. The next article in this series will be devoted to preprocessing, often under-appreciated and underserved, but a crucially important task.
If you’re interested building forecasting systems with impact at scale, apply for a role on our team.
Subscribe to our newsletter to keep up with the latest innovations from Uber Engineering.
Photo Header Credit: The 2009 Total Solar Eclipse, Lib Island near Kwajalein, Marshall Islands by Conor Myhrvold.
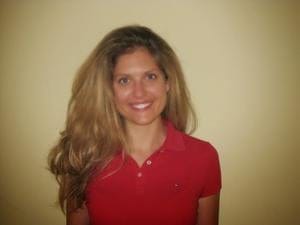
Franziska Bell
Fran Bell is a Data Science Director at Uber, leading platform data science teams including Applied Machine Learning, Forecasting, and Natural Language Understanding.
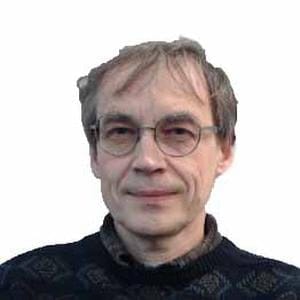
Slawek Smyl
Slawek Smyl is a forecasting expert working at Uber. Slawek has ranked highly in international forecasting competitions. For example, he won the M4 Forecasting competition (2018) and the Computational Intelligence in Forecasting International Time Series Competition 2016 using recurrent neural networks. Slawek also built a number of statistical time series algorithms that surpass all published results on M3 time series competition data set using Markov Chain Monte Carlo (R, Stan).
Related articles
Most popular
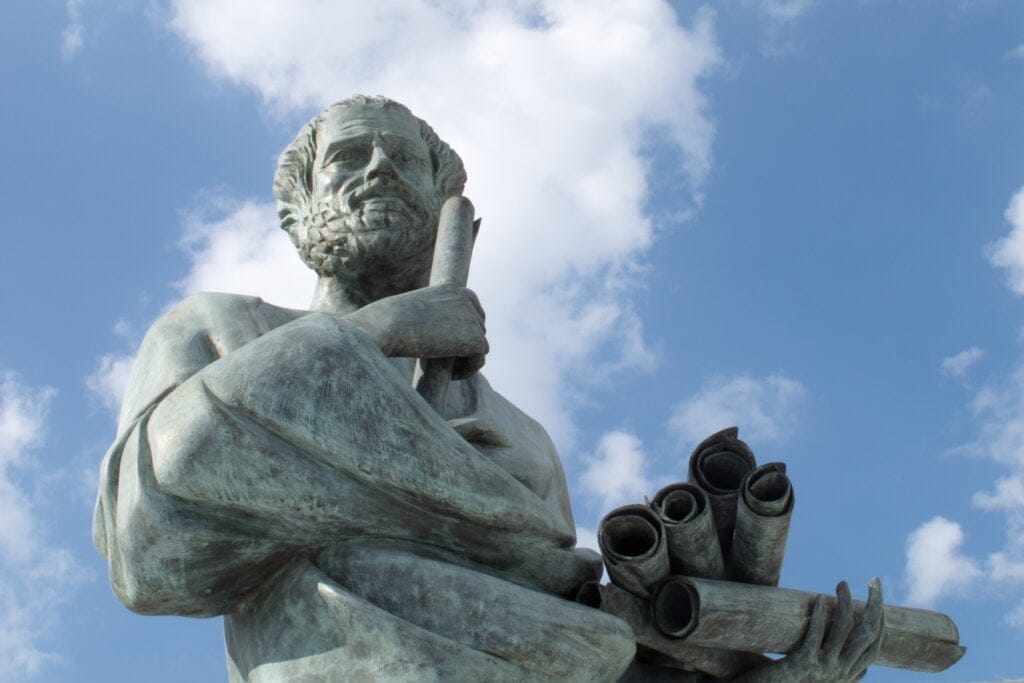
Network IDS Ruleset Management with Aristotle v2
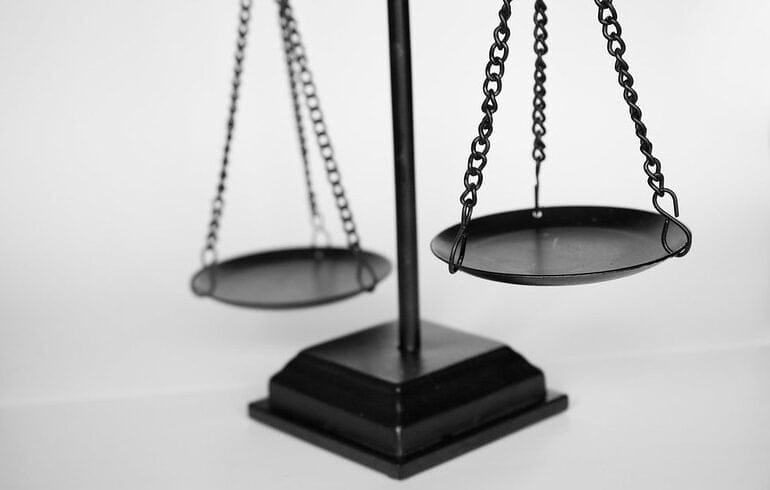
Load Balancing: Handling Heterogeneous Hardware
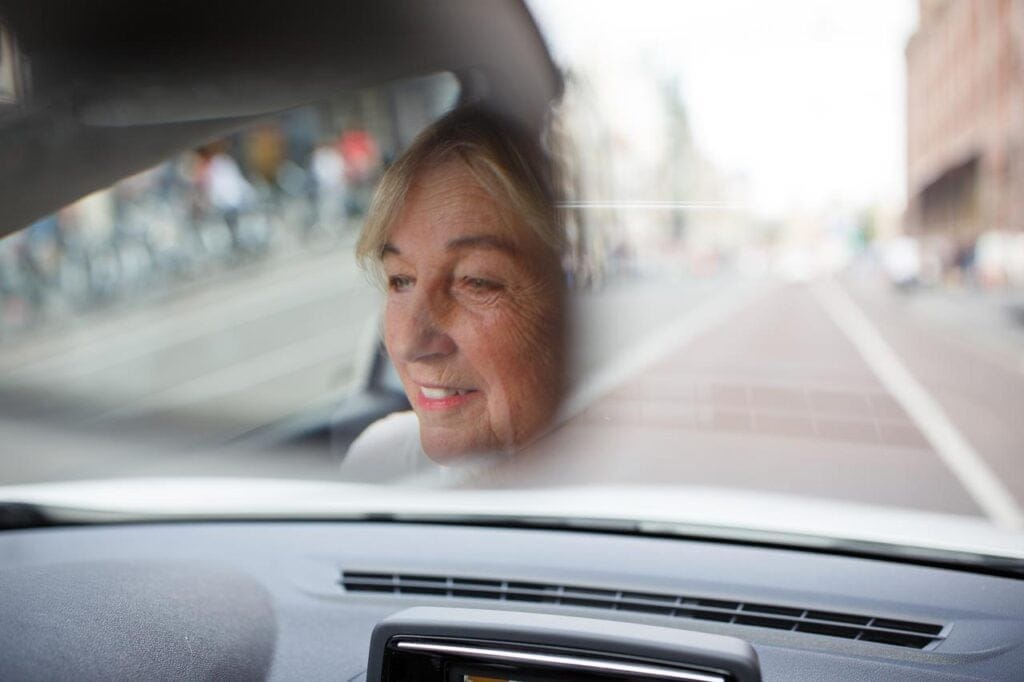
Using Uber: your guide to the Pace RAP Program
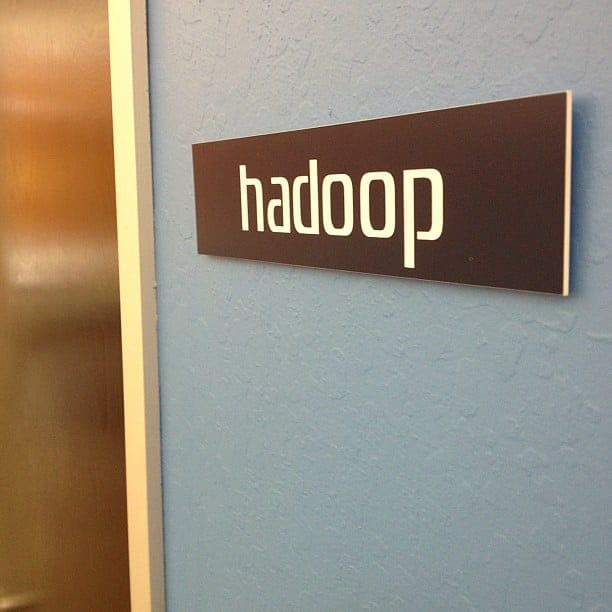
Balancing HDFS DataNodes in the Uber DataLake
Products
Company