Comparing Apples to Googles (to Bings to MapQuests)
Written by
The Science Team is back bringing some #UberData to a topic where there are a lot of opinions but little actual data: maps. Specifically, in this post I compare the accuracy of four major geocoding services: Apple, Google, Bing, and MapQuest.
There was a lot of teeth gnashing and (legitimate) UX/UI design complaints after Apple rolled out their iOS maps. While we can debate about the intelligence of introducing the product when they did and whether or not their UI and places database is effective or complete, what I have yet to see is a statistical analysis of how accurate their geolocation data are. And that’s what’s most important to Uber.
In this post we compare the geocoding accuracy of the following services:
• Apple iOS Location Awareness Geocoding
• Google Maps Geocoding API
• Bing Maps Geocode Service
• MapQuest Geocoding API
Apple put a lot into this move and it cost them. The situation got so out of hand that the head of Apple’s mobile software unit, Scott Forstall, resigned after refusing to apologize for the “Apple maps debacle”. Apple even went so far as to recommend alternatives, giving companies like Waze a huge boost.
Geocoding is a tricky business with a lot of nuances, and Uber doesn’t work without it. As I said over on the O’Reilly Radar blog, as a non-engineer, it’s staggering to think of the complexity of the systems that make Uber work: GPS, accurate mapping tools, a reliable cellular/SMS system, automated dispatching system, and so on. And it’s amazing to think about where some of these everyday, ubiquitous tools come from.
For example, GPS technology arose because physicists William Guier and George Weiffenbach started tracking Sputnik, and were later asked if, instead of tracking a satellite’s position from Earth, if they could track a position on Earth using satellites. Without Sputnik, Uber might not exist today.
Making sure that we know where you are when you request a pickup, and knowing where the nearest driver is to make sure you get a ride quickly is what makes us Uber. Let’s say you’re using our mobile request site to arrange for a pick-up, so you type in your address. How do we find the nearest driver to dispatch your way? First we need to figure out where you are. To do that we need to geocode your address into a latitude and longitude (lat/lng). These two numbers tells us where on the globe you’re located, in the north/south and east/west directions respectively. We can then use this information to determine which driver can get to you most quickly, and the driver then uses the address to find you.
If, instead, you’re using our iOS or Android apps, when you move that pin around the map and request a car we receive your lat/lng. In order for the driver to get your address, however, we need to reverse geocode your lat/lng pair.
The process of going from an address to a lat/lng is relatively straightforward, however the process of going from a lat/lng pair to an address can be a bit more messy. Remember, a lat/lng just tells you where on the globe you’re located. But imagine if you’re living in one of the many places in the Bay Area, for example, where a large house has been converted into multiple independent living units. The ground floor may have one address while the top floor has another. So if you try and reverse geocode the lat/lng into an address which address is correct? The top or bottom unit? There is no one correct answer.
Like in the image below, anywhere along those lines coming off the earth’s surface will have the same lat/lng.
(source)
This is what is known as an “inverse problem”, and is the kind of thing I deal with all of the time in my neuroscience research: if I know which neurons are firing in your brain I can solve the “forward solution” of what the electrical activity would look like when I record it from the surface of your brains. But if all I have is the recording of your brain’s surface electrical activity it turns out that there are an infinite number of possible brain activity states that could give rise to the data I’m seeing.
Similarly, if I know your address I can tell you your lat/lng without a problem (the forward solution). But if all I know is your lat/lng there may be multiple different solutions to your address (the inverse solution… luckily not infinite in this case!)
Okay, let’s get down to the data. In order to compare the geocoding accuracy between Apple, Google, Bing, and MapQuest we need a dataset of known address-to-lat/lng relationships. Thankfully DC.gov has a large database of curated address/geolocation data freely available.
Using this as a starting point, we selected 500 random addresses and geocoded them using each service’s respective API. (The reason we used only 500 is that some of those services have strict API limits, and the Apple geocoding API in particular has no RESTful interface which made doing a large number of queries quite time-consuming.)
Thus for each address, for each service, we get a lat/lng pair. We can then calculate how far away from the known location this geocoded lat/lng pair is. Now remember we’re only doing this for 500 addresses for 1 US city, but this should be sufficient to get an error estimate.
The first result to jump out is:
Apple, Google, and Bing all show that 80%+ of the geolocated points are within 25 meters of the actual location. MapQuest, on the other hand, fares less well with only 36% of the locations within 25 meters.
This discrepancy can be see when we plot the median error for each service:
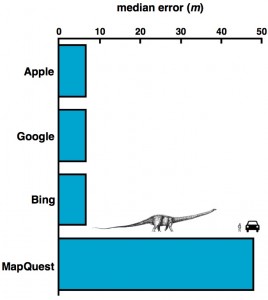
That’s a big gap. Apple, Google, and Bing all have essentially similar error rates, with the average and median errors around 7-9 meters. That’s not too bad! MapQuest, on the other hand, has error rates around 50 meters. That’s less not bad. 50 meters is a lot of room… it gives you plenty of room to jump in your Uber car before one of the longest dinosaurs to have ever existed can come stomp you.
What’s interesting is looking at the histogram of MapQuest’s errors:
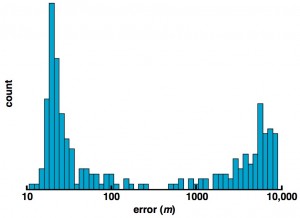
This distribution is bimodal! It’s got a big cluster around a relatively low error of around 20-30 meters, but then another cluster with a much larger error of several kilometers! This looks very different from the error distributions of the other services. Here’s Apple, with a nice unimodal low error:
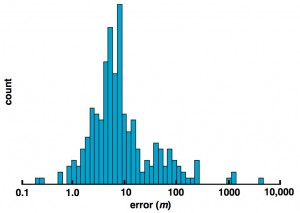
What’s more, even if the address/geolocation mapping in the test dataset of DC addresses is incorrect, we can still get a feel for the fidelity of the different providers by looking at the correlations between their errors. So even if the test data isn’t 100% perfect, the different providers should be giving similar errors if they’re actually locating the real location of the address. Which, as you can see below, is true.
For Apple, Bing, and Google (click the image for a sharper view; x and y axes are in units of log_10 error in meters):
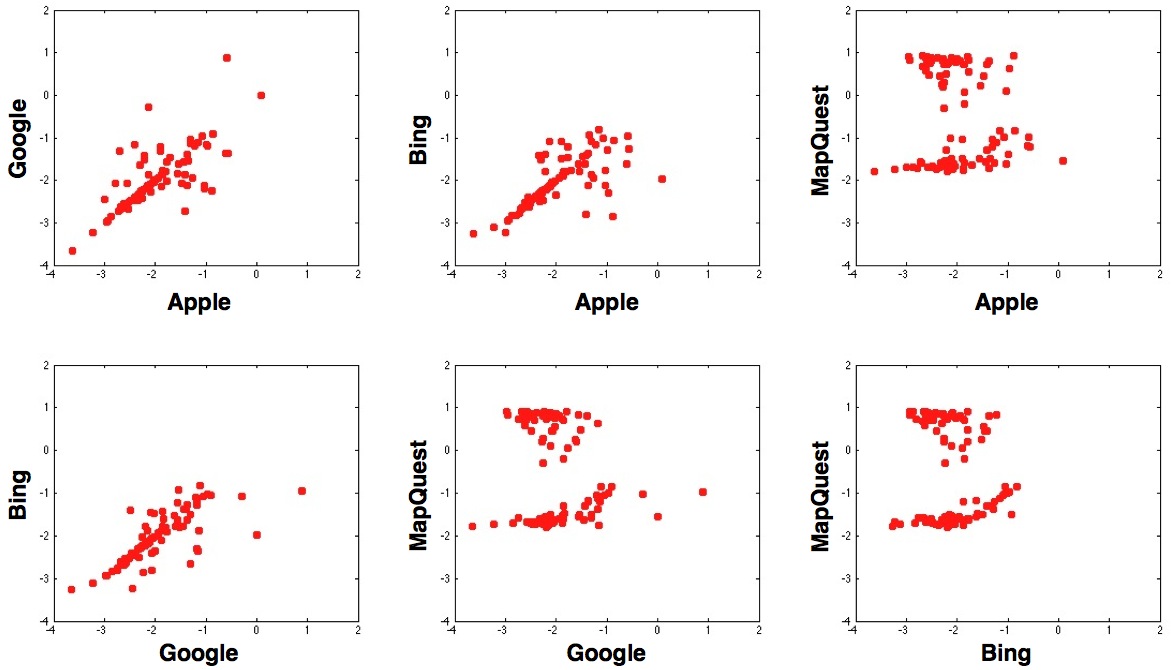
But it looks like MapQuest has some fundamentally different data from the other providers. Totally strange responses. What’s the deal?
Well, the addresses in the database I used are formatted:
XXXX ANYSTREET RD YY, WASHINGTON, DC
where XXXX is the street number and YY is a cardinal direction: NE, SE, SW, or NW. Apparently MapQuest’s geocoding API does not like those cardinality pointers.
Here’s what happens to their error if I just remove the cardinality information entirely:
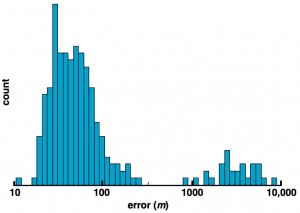
Much better! That results in a drastic reduction of the overall error. For example, previously the address with the most error was located 9.06km away from its actual location in MapQuest. After removing the “NW” from the address it dropped to 20 meters.
So what does this tell us? Overall, most of the services perform quite well (including Apple). So Apple Maps don’t seem to have an accuracy issue, at least.
Of course there are several caveats! This is a very low sample size for data limited to one US city. Caveat lector! But the correlations between errors, as well as the error distributions, are informative.
From a data science perspective, hammering your own system with queries and comparing the results to known locations seems like an obvious performance test to figure out your own weaknesses. Given how much cardinal directions in an address break MapQuest, I’d guess they’ve not done this.
I’m curious as to why these cardinal coordinates cause MapQuest’s accuracy to drop so much, but the couple of sanity-checks we performed didn’t yield any obvious answers.