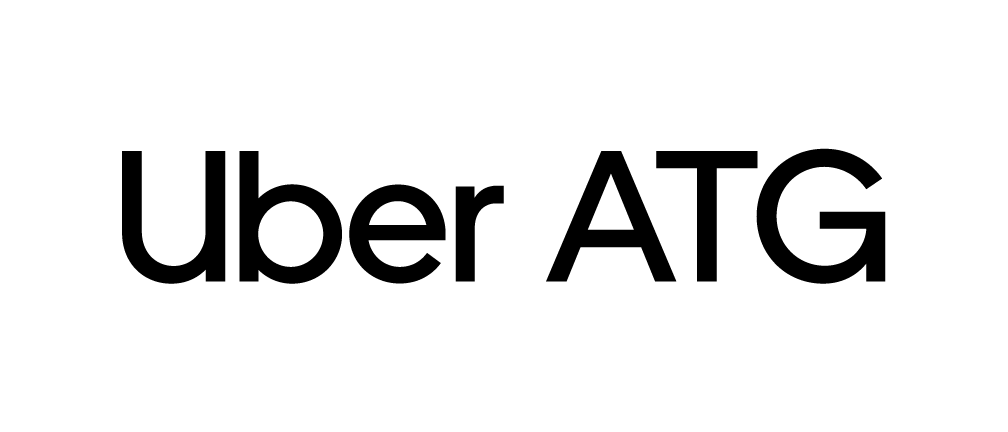
Uber ATG is committed to publishing research advancements with the goal of bringing self-driving cars to the world safely and scalably. We hope our approach to sharing will deepen the interactions and collaborations between industry and academia, and will ultimately bring self-driving research communities together.
This fall, the International Conference on Computer Vision (ICCV), the Conference on Robot Learning (CoRL), and the Intelligent Robots and Systems (IROS) conference will be taking place in Asia. Uber ATG’s research scientists will be participating in these conferences in oral presentations, posters and workshops.
This year, Uber ATG has five publications accepted at ICCV, two publications accepted at CoRL, and two publications accepted at IROS. In addition, Raquel Urtasun, Uber ATG Chief Scientist and Head of Uber ATG R&D, will be giving four talks at ICCV.
We encourage you to interact with us during these events. For the full schedule of Uber ATG’s presence at ICCV, check out our official event page.
Read on to learn about our presence at these conferences and our new state-of-the-art research:
Uber ATG at ICCV 2019
We will be located at Booth # D-7.
Namdar Homayounfar, Wei-Chiu Ma*, Justin Liang*, Xinyu Wu, Jack Fan, and Raquel Urtasun
When: Tuesday, October 29, 2019 at 3:30 p.m. KST; Hall B
Summary: We map complex lane topologies in highways by formulating the problem as a deep directed graphical model, where the nodes of the graph encode geometric and topological properties of the local regions of the lane boundaries. We demonstrate the effectiveness of our approach on two major North American highways in two different states, and show high precision and recall as well as 93 percent correct topology.
Jerry Liu, Shenlong Wang, and Raquel Urtasun
When: Wednesday, October 30, 2019 at 9:36 a.m. KST; Hall D2
Summary: We design a novel autoencoder-based architecture for compressing a stereo image pair that extracts shared information from the first image in order to reduce the bitrate of the second image. We demonstrate a 30-50 percent reduction in the second image bitrate at low bitrates.
Xiaohui Zeng, Renjie Liao, Li Gu, Yuwen Xiong, Sanja Fidler, and Raquel Urtasun
When: Wednesday, October 30, 2019 at 10:30 a.m. KST; Hall B
Summary: We propose the differentiable mask-matching network (DMM-Net) to solve the video instance segmentation problem where the initial instance mask is provided. On DAVIS 2017 data set, DMM-Net achieves the best performance without online learning on the first frame and the second best with it. Without any fine-tuning, DMM-Net performs comparably to state-of-the-art methods on the SegTrack version two data set.
Shivam Duggal, Shenlong Wang, Wei-Chiu Ma, Rui Hu, and Raquel Urtasun
When: Wednesday, October 30, 2019 at 10:30 a.m. KST; Hall B
Summary: We propose a real-time dense depth estimation approach using stereo image pairs, which utilizes differentiable Patch Match to progressively prune the stereo matching search space. Our model achieves competitive performance on the KITTI benchmark while being real time.
Yun Chen, Bin Yang, Ming Liang, Raquel and Urtasun
When: Friday, November 1, 2019 at 3:30 p.m. KST; Hall B
Summary: We design a simple yet effective architecture that fuses information between 2D and 3D representations at multiple levels. We show state-of-the-art results on the KITTI depth completion benchmark.
ICCV – Workshop on Large Scale Holistic Video understanding
Invited speaker: Raquel Urtasun
When: October 27, 2019, 14:10-14:40 PM KST
Where: The COEX Convention Center, Seoul, Korea 308BC
ICCV – Visual Perception for Navigation in Human Environments
Invited speaker: Raquel Urtasun
When: October 28, 2019, 9:20-9:50 AM KST
Where: The COEX Convention Center, Seoul, Korea 307 A
ICCV – Workshop on Autonomous Driving
Invited speaker: Raquel Urtasun
When: October 28, 2019, 10:45-11:15 AM KST
Where: The COEX Convention Center, Seoul, Korea, 401
ICCV – Autonomous Navigation in Unconstrained Environments (AutoNUE)
Invited speaker: Raquel Urtasun
When: November 2, 2019, 11:00-11:45 AM KST
Where: The COEX Convention Center, Seoul, Korea, E1
Uber ATG at CoRL 2019
Ajay Jain, Sergio Casas, Renjie Liao, Yuwen Xiong, Song Feng, Sean Segal, and Raquel Urtasun
When: Wednesday, October 30, 2019 at 11:00 a.m. JST
Summary: Our research shows that non-parametric distributions can capture extremely well the (erratic) pedestrian behavior. We propose Discrete Residual Flow, a convolutional neural network for human motion prediction that accurately models the temporal dependencies and captures the uncertainty inherent in long-range motion forecasting. In particular, our method captures multi-modal posteriors over future human motion very realistically.
Kelvin Wong, Shenlong Wang, Mengye Ren, Ming Liang, and Raquel Urtasun
When: Thursday, October 31, 2019 at 3;30 pm. JST
Summary: We propose a novel open-set instance segmentation algorithm for point clouds that identifies instances from both known and unknown classes. In particular, we train a deep convolutional neural network that projects points belonging to the same instance together in a category-agnostic embedding space.
Uber ATG at IROS 2019
Abbas Sadat*, Mengye Ren*, Andrei Pokrovsky, Yen-Chen Lin, Ersin Yumer, and Raquel Urtasun
When: Wednesday, November 6, 2019 at 1:30 p.m. CST; Room LG-R15
Summary: We propose a novel method to jointly learn the linear weights of the interpretable cost functions of behavior planning and trajectory generation from human demonstrations. Experiments on real-world self-driving data demonstrate that the jointly learned planner performs significantly better compared to baselines that do not adopt joint behavior and trajectory learning, under certain circumstances.
Wei-Chiu Ma*, Ignacio Tartavull*, Ioan Andrei Barsan*, Shenlong Wang*, Min Bai, Gellért Máttyus, Namdar Homayounfar, Shrinidhi Kowshika Lakshmikanth, Andrei Pokrovsky, and Raquel Urtasun
When: Wednesday, November 6, 2019 at 5:45 p.m. CST; Room LG-R8
Summary: We propose a novel semantic localization algorithm that exploits multiple sensors and has precision on the order of a few centimeters. Our approach does not require knowledge about the appearance of the world, and our localization maps take orders of magnitude less storage when compared to the maps utilized by traditional geometry and intensity-based localizers.
Please come visit us at ICCV (booth #D-7) IROS and CORL to learn more about our lab’s research, discuss the work with our researchers, and hear about career opportunities with Uber ATG.
Not attending these conferences? Check out Raquel Urtasun’s recent joint keynote with Eric Meyhofer, Head of Uber ATG, during the 2019 Uber Elevate Summit to learn how her team’s research is powering the future of self-driving cars:
We look forward to seeing you there!
Interested in working on self-driving cars? Learn more about research opportunities with Uber ATG by visiting our careers page.
Author names followed by a “*” indicate non-Uber affiliations.
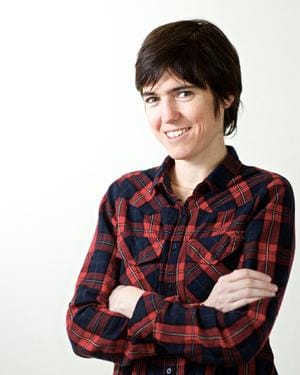
Raquel Urtasun
Raquel Urtasun is the Chief Scientist for Uber ATG and the Head of Uber ATG Toronto. She is also a Professor at the University of Toronto, a Canada Research Chair in Machine Learning and Computer Vision and a co-founder of the Vector Institute for AI. She is a recipient of an NSERC EWR Steacie Award, an NVIDIA Pioneers of AI Award, a Ministry of Education and Innovation Early Researcher Award, three Google Faculty Research Awards, an Amazon Faculty Research Award, a Connaught New Researcher Award, a Fallona Family Research Award and two Best Paper Runner up Prize awarded CVPR in 2013 and 2017. She was also named Chatelaine 2018 Woman of the year, and 2018 Toronto’s top influencers by Adweek magazine
Posted by Raquel Urtasun
Related articles
Most popular
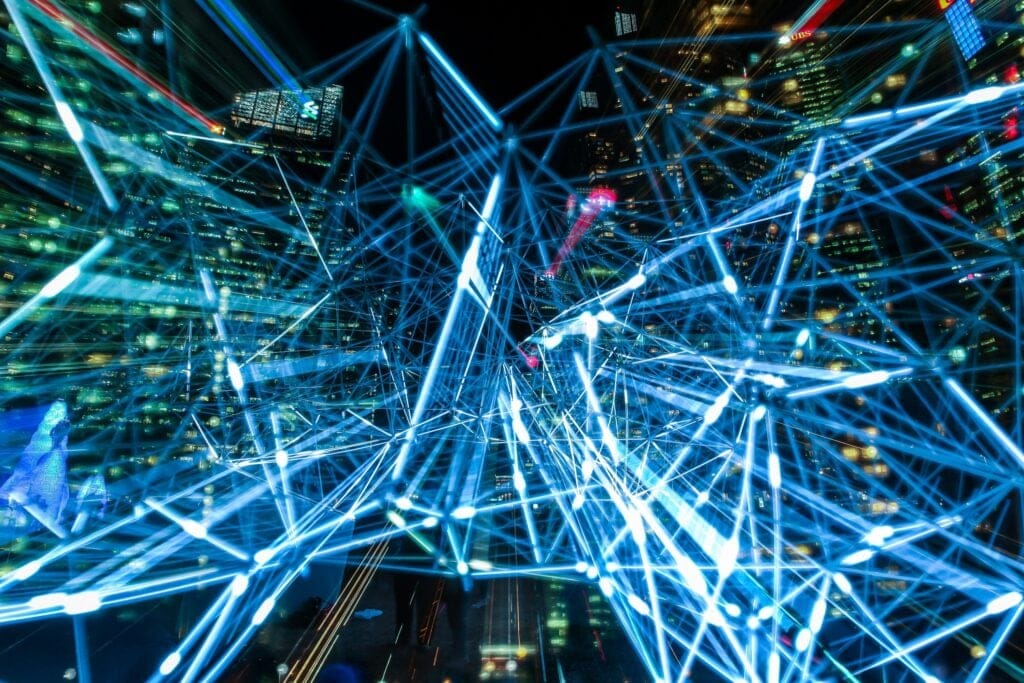
Jupiter: Config Driven Adtech Batch Ingestion Platform
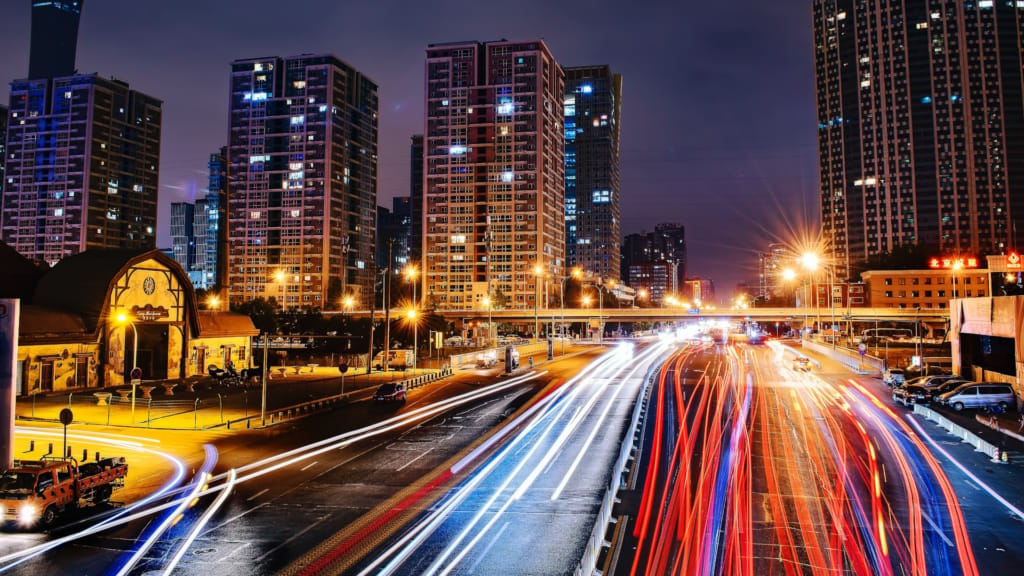
How Uber Serves Over 40 Million Reads Per Second from Online Storage Using an Integrated Cache
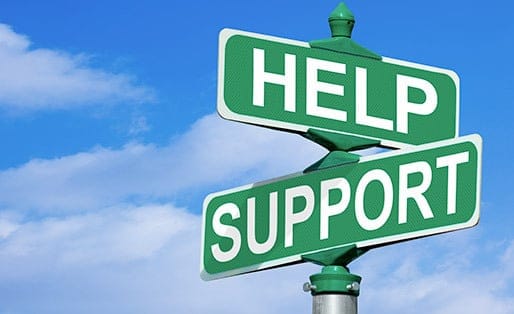
Building Scalable, Real-Time Chat to Improve Customer Experience
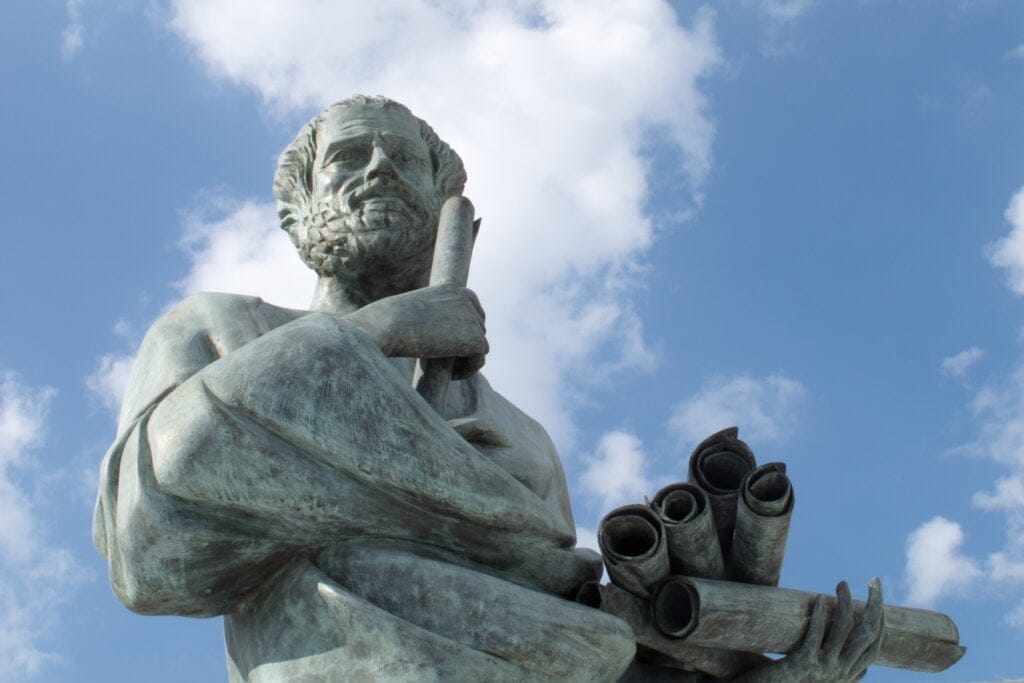
Network IDS Ruleset Management with Aristotle v2
Products
Company