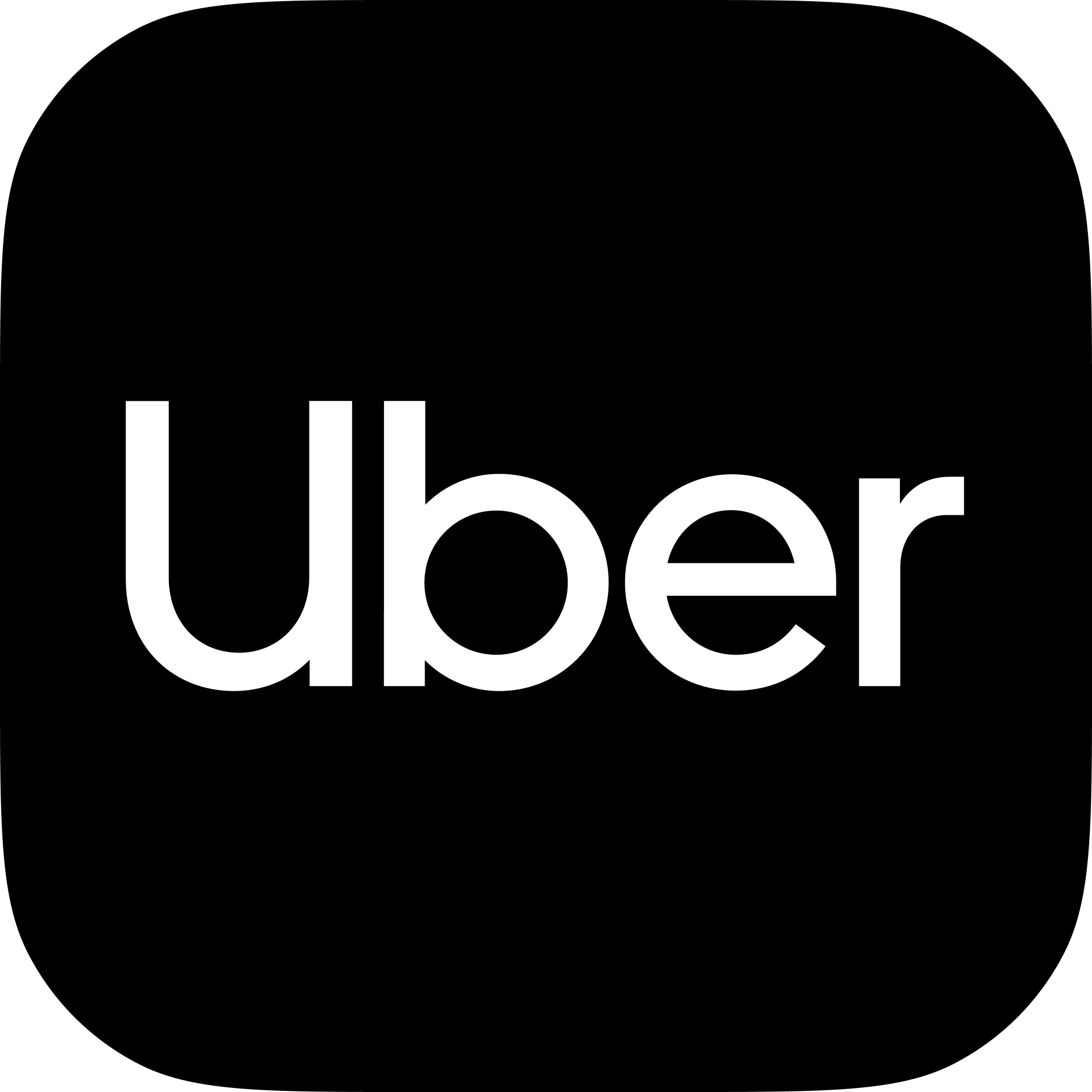
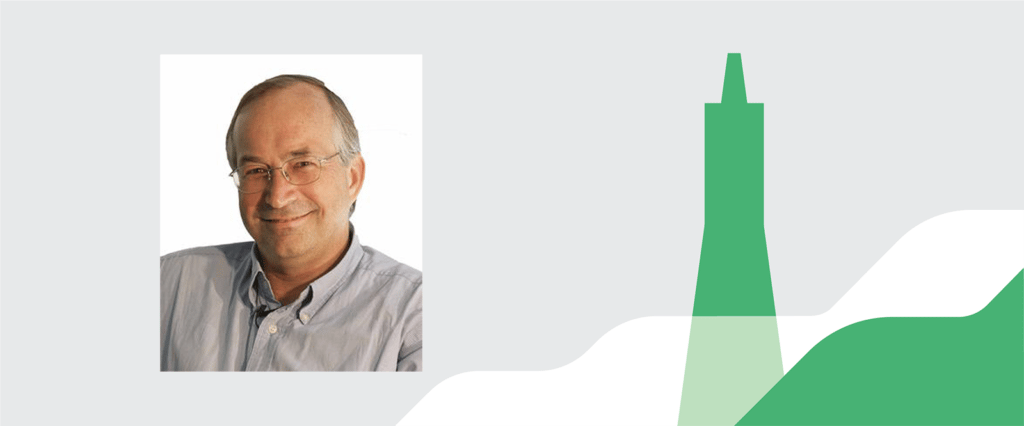
Whenever a rider gets dropped off at their location, one of our driver-partners finishes a session laden with trips, or an eater gets food delivered to their door, data underlies these interactions on the Uber platform. And our teams could not serve these interactions efficiently without an impressive roster of people experienced in machine learning and AI.
To this point, we are delighted to welcome Jan Pedersen to Uber AI. Jan has a tremendous amount of experience in technology and science leadership roles, having served as Chief Scientist for Core Search at Microsoft, VP for Data Science at Twitter, and most recently, Chief Scientist at eBay. He joins Uber as a Distinguished Scientist and will help us navigate and grow our AI and machine learning efforts.
We sat down with Jan to get some insight into his past experience and his decision to join Uber:
What drew you to study statistics?
When I entered undergraduate at Princeton, I wanted to study physics, but I realized fairly quickly that computer science (CS) really interested me. However, at that time CS was in the engineering school, and I was enrolled in the school of arts and sciences. Changing schools would have required an extra year of study, which I couldn’t inflict on my parents. Instead, I looked for a science major that offered maximum flexibility, which turned out to be statistics. Although initially a matter of convenience, I grew to really like the field. At its core, statistics is about the methodology of science, making it quite fascinating. It didn’t hurt that at Princeton I was exposed to some of the great minds in the field, such as John Tukey.
An early part of your career was spent at Xerox PARC, a company that generated a number of revolutionary technologies. What was it like to work there, and what projects did you work on?
I was a member of one of the AI labs at PARC, which focused on InterLisp-D and expert systems. I was in the Natural Language Processing (NLP) group.
I began at PARC as an intern while completing my PhD in statistics at Stanford. For my doctorate, I implemented a programming environment for data analysis in Lisp called Interactive Data Language. This work gave me the credentials to join the Lisp systems group at PARC, which was focused on commercializing the Interlisp_D Lisp Machine, a personal computer with an integrated programming environment microcoded for Lisp. I implemented various aspects of the Lisp operating system, including the arithmetic and sequence function libraries as well as the package system.
I graduated into the research team and joined the NLP group, where I focused on corpus-based computational linguistics and wrote a number of frequently referenced early papers on topics such as Markov model part-of-speech tagging, text categorization, document clustering, and document summarization. With a good friend and colleague of mine, Doug Cutting (who is well-known in the open source community as an author of Lucene and Hadoop), I wrote a text retrieval system in Lisp called Text Database (TDB), which was a great introduction to search technology. The development of TDB coincided with the first wave of web search engines, such as Lycos, Excite, Alta Vista, and InfoSeek, and opened up opportunities for me outside of PARC.
During your career, Internet use grew substantially, and has become a huge part of society. How did your professional application of statistics change as a result of internet and data growth?
Every great internet business is built on the intelligent use of data, including the ability to experiment at a fine grain and identify patterns in usage data. Early internet companies were perhaps slow to come to this realization, but now it is an established fact. I was fortunate to have a combination of skills useful in this environment. In particular, knowledge of statistics is essential to fully appreciate experimentation, which is (or should be) at the heart of internet development cycles. Similarly, predictive modeling, or machine learning, is a wonderful way to extract value from data.
Given your experience working with internet search projects, how has this specific area changed over the years?
It’s difficult to realize how much search has improved because the evolution has been gradual, but if you were to compare a search engine from five years ago to today, you would appreciate the difference. Perhaps the biggest change has been the move from returning a list of relevant documents, the classic 10 blue links, to returning an actual answer. The answer might come from a knowledge graph, or it might be extracted from a document. The NLP technology required to analyze a query is quite demanding, but getting it right sets you up for answering general questions, which you see now on prominent display in speech-only home assistants, such as Google Home and Amazon Alexa.
How has machine learning impacted your work?
I’ve used machine learning throughout my career. My early work was all about applied machine learning to NLP tasks, such as part-of-speech tagging. At Alta Vista, we were the first search team to deploy machine-learned ranking at scale using gradient boosted trees. The entire search stack at Microsoft Bing was based on machine learning. It really is a fantastic tool with an incredible range of applications.
Why did you make the decision to join Uber?
Uber is a fascinating company that will make a positive impact on the world. It has become an essential service that makes our lives better with a seemingly limitless horizon of potential new business and applications.
Uber is also well-regarded in the industry for tech innovation and a commitment to AI. I see many interesting opportunities at Uber AI Labs since AI and machine learning are so fundamental to Uber’s business. I’m still on a learning curve, but look forward to focusing on joint projects with key Uber AI partners in the near future.
Interested in working with Jan and Uber AI Labs? Consider applying for a role on our team!
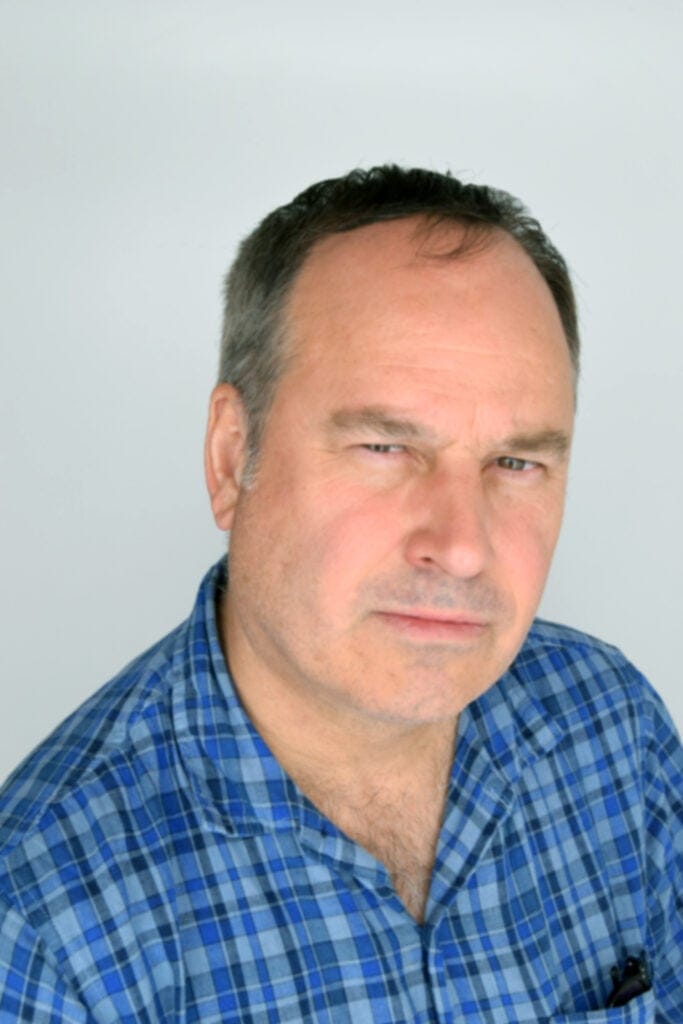
Wayne Cunningham
Wayne Cunningham, senior editor for Uber Tech Brand, has enjoyed a long career in technology journalism. Wayne has always covered cutting edge topics, from the early days of the web to the threat of spyware to self-driving cars. In his spare time he writes fiction, having published two novels, and indulges in film photography.
Posted by Wayne Cunningham
Related articles
Products
Company