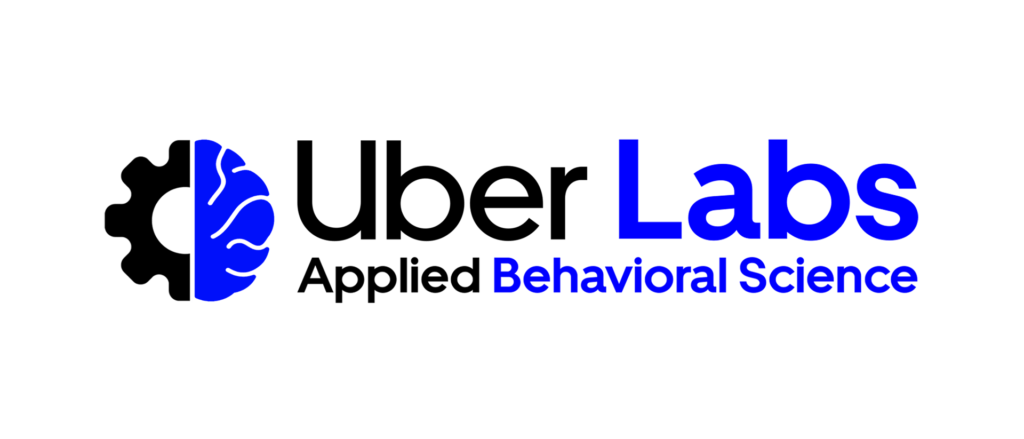
On the Uber Labs team, our mission is to leverage insights and methodologies from behavioral science to build programs and products that are intuitive and enjoyable for customers. Our group of scientists have PhDs in fields including psychology, marketing, and cognitive science, with domain knowledge in topics including decision making, motivation, and learning, methodological capabilities in experimental and quasi-experimental design, and statistical prowess in areas such as hierarchical modeling and causal inference approaches. With our domain expertise, we provide insight into topics like how to increase customer satisfaction, and with our methodological and statistical expertise, we provide answers to questions like how to quantify the business impact of customer satisfaction (with mediation modeling being one such approach).
In this article, we delve into our team’s approach to applying theoretical insights from behavioral science, exploring why and how we partner with product and marketing teams to build better products and experiences for our customers. Specifically, we highlight an example in which we used theoretical insights around perceptions of waiting and time to improve riders’ experience of a newly launched product, Express POOL.
Leveraging insights from academia
Behavioral science is a generic term that, similar to the term data science, encompasses a broad spectrum of potential roles and may be applied differently across companies. At Uber Labs, we define behavioral science as the study of how people think and behave. We include many fields in our concept of behavioral science, such as psychology, behavioral economics, sociology, political science, and neuroscience. Researchers within these fields have spent decades conducting rigorous empirical research to discover principles around how and why people behave the way that they do. People are complex, so behavioral scientists have developed diverse toolkits; they include novel methodological and statistical approaches to handling complex issues, like not always being able to run randomized controlled experiments. We can utilize these theoretical insights and methods in industry settings.
When collaborating with Uber’s product teams, the Uber Labs team typically provides end-to-end support, beginning with applying our domain expertise to problems by providing recommendations based on theory, and then by supporting the execution and analysis of the planned approach (for instance, conducting experiments). Our working model differs from what other behavioral scientists may be doing in industry; for example, at consultancies, behavioral scientists may focus exclusively on applying domain expertise, and in other organizations, individuals trained in behavioral science may focus on methodology and statistics, running and analyzing experiments or building surveys. Our end-to-end approach stems in part from our origins as the experimentation team for the operations side of the business.
The road to becoming behavioral (data) scientists
In 2014, Uber was launching our ridesharing app in new cities around the world on a near-daily basis. Local operations teams in every region needed to understand which communications and products worked best in their local contexts, but most of them lacked a background in experimental design and statistics. To serve these needs, we formed Uber Labs, a team of scientists with backgrounds in fields including psychology, marketing, and cognitive science. This centralized team set out to apply its methodological capabilities in experimental and quasi-experimental design and statistical expertise in areas such as hierarchical modeling to enhancing our products for the benefit of riders and driver-partners in diverse regions.
While one-on-one consulting was effective, we needed to scale this expertise across our ever-growing product stack. By building self-serve sample size and statistical analysis calculators with Shiny and an R package, we empowered non-technical teams to leverage our expertise. These tools, which simply required users to upload their raw data, included built-in checks for statistical assumptions and model fit, as well as an automated flow which selected the appropriate analytic method for a given dataset; on the user’s end, the output showed both the analysis results and plain language explanations. We later worked with the experimentation platform team in building the analysis flow and data checks for our A/B test analysis tool, making it even simpler for teams to conduct appropriate analyses.
As the company grew and expanded into more diverse product areas, we saw an opportunity to increase our impact by partnering directly with product teams. In early 2017, we expanded our mission to focus on applied behavioral science in addition to statistics. We shifted from being reactive in providing support for pre-formulated ideas to proactive in leveraging our domain backgrounds in areas such as learning and memory, which allowed us to propose solutions based on existing scientific insights. We also began supporting new areas in addition to experimentation: product strategy, program design, content optimization, and measurement of business impact.
Through our training, behavioral scientists are well-versed in both qualitative and quantitative research. At the time when we expanded our mission, we also changed roles from being research scientists to data scientists, choosing to focus on quantitative research as part of the data science function. Uber has a top-notch UX research team which conducts qualitative research. Focusing on quantitative applications of behavioral science, such as testing theory-based ideas through experiments and applying novel statistical approaches, enables us to complement Uber’s broader research ecosystem.
Our workflow: how we deliver insights and methods
We have developed a workflow that allows us to deliver immediate impact via consultations as well as long-term efficiency by scaling behavioral science insights and methods through templates and platforms. Expanding on these three components:
- Consultations are the most efficient approach for tactical problem solving at the level of a specific product or feature. We work directly with product, marketing, and other data science teams to provide behavioral science-based recommendations for the challenges they face.
- To inform product and analytic strategy more broadly, our team develops guidelines for areas like content creation and program development and templates like R and Python analysis packages, empowering internal customers at Uber to learn and replicate our approaches independently.
- Finally, we partner with platform teams across the company to provide our customers with click-of-a-button access to our insights and methodologies. Our work with the experimentation platform team on the post-experiment analysis tool serves as an example.
Many of our consultations involve applying theoretical insights to problems, which we will describe in an example below. Additionally, as behavioral data scientists we take a quantitative approach to problem solving. Our data work focuses on questions about user behavior and falls into three categories: quantifying psychological constructs and processes, applying behavioral science methods, and conducting experiment analysis.
First, we use Uber data to quantify latent psychological constructs and processes that drive behavior. To do so, we develop novel techniques or adapt existing methods from the social and behavioral sciences, such as factor analysis. Next, we apply methods less commonly used in data science to solve otherwise intractable problems. Examples include mediation modeling and causal inference approaches like interrupted time series analysis. Finally, we analyze data from experiments, ranging from standard A/B tests to approaches used when A/B tests are not possible or recommended, like randomized encouragement designs.
One aspect of the shift to industry from academia that members of our team have found exciting has been the ability to work use applied research to improve user experiences. In academia, most of us focused on research with the aim of advancing theory, rather than solving specific problems.
As part of this focus on application, one issue that we noticed after starting to consult with product teams on behavioral science is that concepts may seem easy to understand and apply, but indiscriminate application can lead to unanticipated consequences; the situational and individual context need to be considered. For example, one behavioral science concept many people are familiar with is loss aversion. The concept seems straightforward: people are generally more motivated to avoid losses than acquire gains. However, there are many situations in which framing something as a loss could frustrate or annoy a user rather than motivate. For instance, a longtime user of a rewards program who has been accustomed to earning points, with points accrual as her only experience with the program, may become angry if threatened with loss of points if she fails to make a quick purchase. Even general tendencies like loss aversion may have unintended negative consequences if used without considering the context. Regardless of the expected success of a treatment, we recommend experimentation to better understand its impact.
Use Case: Express POOL
Because behavioral science is highly contextual, most of our work transpires through consultative partnerships with teams. Our partnership with the Express POOL team provides an example of how the Applied Behavioral Science team applies theories from academic research to product development.
In early 2018, Uber launched Express POOL. Similar to uberPOOL, Express POOL involves sharing a ride with other riders who are headed in the same direction. Unlike uberPOOL, uberX, and our other ridesharing products, Express POOL includes extra wait time between requesting and matching with a car and a short walk to their pick-up location; these changes create straighter, more efficient routes, in turn leading to a more affordable trip.
Riders were accustomed to quicker matching and a door-to-door experience, so the product team was paying close attention to riders’ use of the new product. The team noticed that there was room for improvement: riders were cancelling their trips in the time between request and matching—a period that included the extra wait time—at a high rate relative to other products.
In our typical consultation workflow, we begin by meeting with collaborators from a partner team to understand the problem at hand. This team usually includes a product manager, product marketing manager, user experience researcher, engineer, and product data scientist. We consider prior research from the team, such as readouts from usability testing. In this case, when we joined the project, we learned the details described above about how riders were responding to the new Express Pool product.
Having learned the context for the problem at hand, our first step was to conduct a literature review, or in other words, a behavioral science deep dive, to identify the relevant theories and insights to address a given problem. With this deep dive, we translate the academic literature into concrete applications for the product team and recommend ways to test these concepts in the product.
In this case, we dove into the behavioral science literature to gather insights about people’s perceptions of time and waiting. We identified three concepts that are important in presenting wait time: idleness aversion, operational transparency, and the goal gradient effect. The concept of idleness aversion is face valid: people dread idleness and want to be busy. Operational transparency, or revealing to consumers what is going on under the hood, so to speak, has been found to increase consumer’s valuation of products. Finally, the goal gradient effect describes the greater motivation and effort exerted in goal pursuit when people feel like they are advancing well towards their objective.
Given these insights, we recommended highlighting progress during wait times by explaining each granular step going on behind the scenes, like identifying other riders traveling the same way and finding a car for the trip. Additional information—for example, explaining the arrival time estimate calculation—could be provided by clicking an info icon. The Express POOL team tested these ideas in an A/B experiment and observed an 11 percent reduction in the post-request cancellation rate.
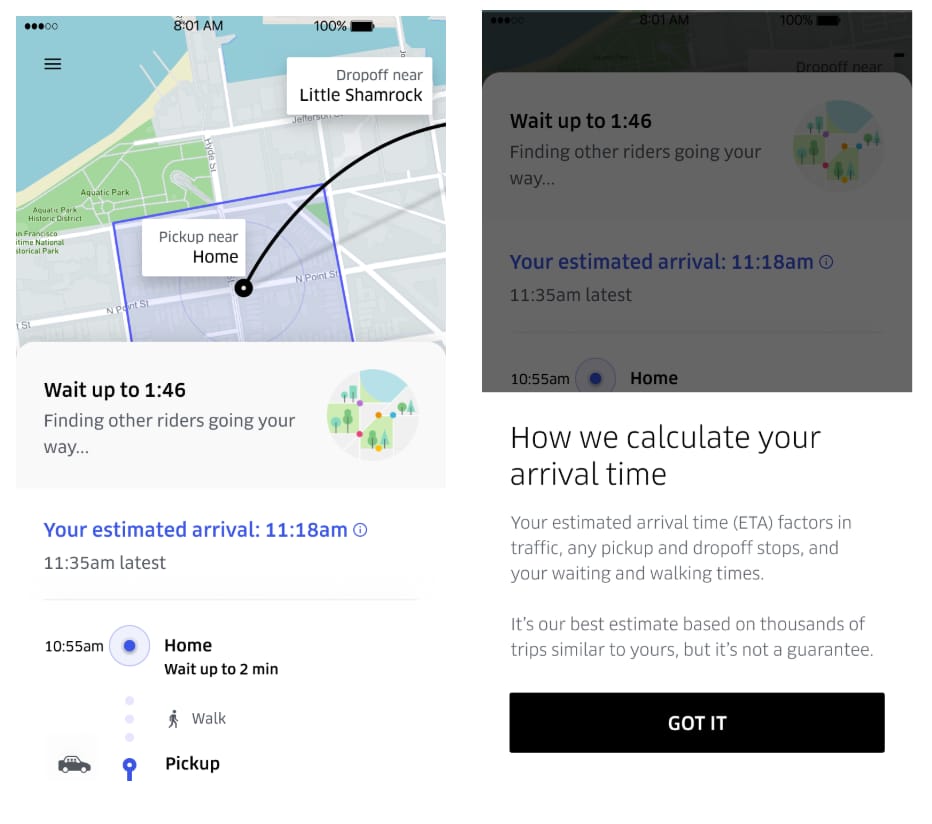
As described in this example, after conducting the behavioral science deep dive, we worked with the partner team to prioritize ideas based on estimated impact and potential risks. We then led experiments to test our proposed ideas, starting from experiment design through analysis. The entire applied behavioral science project workflow, as embodied by our work on Express POOL, is depicted in Figure 2, below:
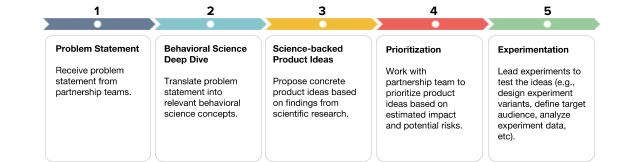
Behavioral science adds value
Our work on Express POOL demonstrates the unique value that we provide as behavioral scientists with a perspective on the product backed by decades of scientific research. Armed with these insights, we work alongside UX researchers and product data scientists who use their skills to tackle a different set of problems from the issues that we investigate. For instance, during our Express POOL experiment, product data scientists carefully monitored the health metrics for products and noticed room for improvement in the post-request cancellation rate. UX researchers conducted ride-along trips to understand riders’ pain points and hone in on the problem. As behavioral data scientists, we leveraged our domain and methodological expertise to suggest an empirically testable solution to that problem.
We account for our specialized skill set and how we can add value when we select and scope out partnerships and projects. At a high level, we consider the priorities for the year set by product leadership in terms of business impact. At a more granular level, when prioritizing the projects that we will do within a partnership, the product team provides input into which areas have the strongest business need. Importantly, our team views these areas with a behavioral science lens, determining whether or not our domain knowledge and quantitative expertise will be utilized. In some cases, this could mean deprioritizing analysis for experiments which lack a strong theoretical basis or purely qualitative research which does not require our methodological skills. Maximizing for both business impact and behavioral science relevance allows our team to have the biggest impact.
Key takeaways
Looking forward, as Uber expands into new modalities and refines existing products, we anticipate many opportunities for our team to use behavioral science to provide the best possible experience for our users. In 2019, we will continue to partner with other teams on innovative, high-impact projects as well as invest in efforts to scale our insights to make behavioral science accessible. We are excited to further leverage a workflow that utilizes our expertise, in regard to both theoretical insights and methods, and adds value to the features, programs, and platforms being built across our company.
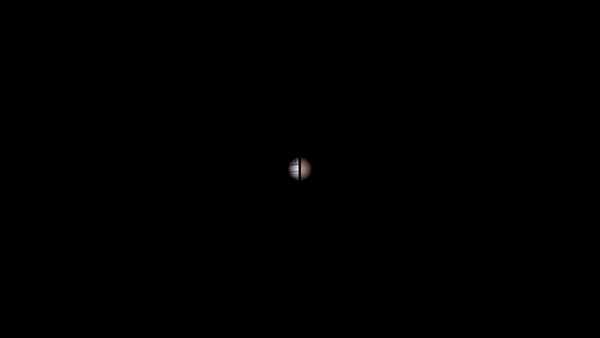
Interested in applying behavioral science to product and marketing problems? Consider applying for a role on our team! We have openings in San Francisco and Amsterdam, as well as an internship in San Francisco.
Header image attribution: the gear icon in the Uber Behavioral Science logo is from Gear Vector Drawing created by Ahkâm and hosted on Free Icons Png.
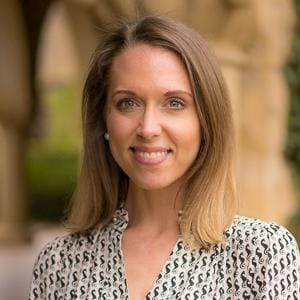
Candice Hogan
Candice Hogan is a data science manager leading Uber Labs, the Applied Behavioral Science team.
Posted by casey.flint@uber.com, Candice Hogan