Model Excellence Scores: A Framework for Enhancing the Quality of Machine Learning Systems at Scale
21 March / Global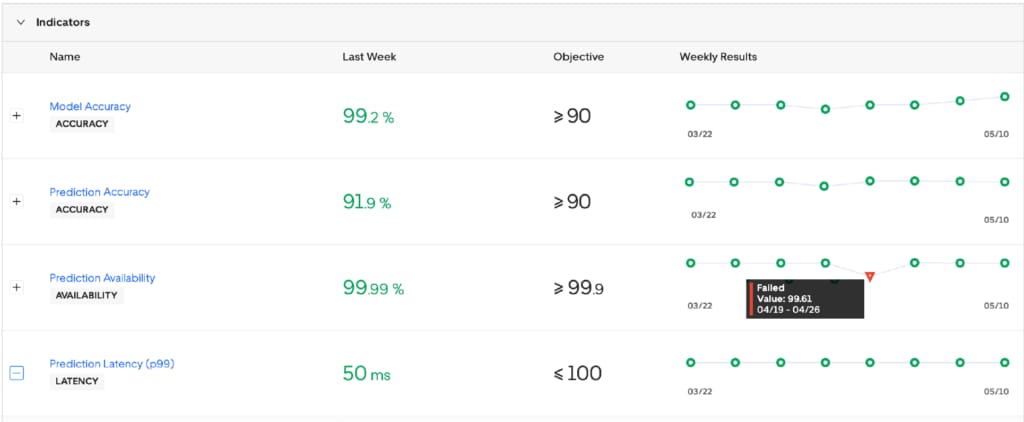
Introduction
Machine learning (ML) is integral to Uber’s operational strategy, influencing a range of business-critical decisions. This includes predicting rider demand, identifying fraudulent activities, enhancing Uber Eats’ food discovery and recommendations, and refining estimated times of arrival (ETAs). Despite the growing ubiquity and impact of ML in various organizations, evaluating model “quality” remains a multifaceted challenge. A notable distinction exists between online and offline model assessment. Many teams primarily focus on offline evaluation, occasionally complementing this with short-term online analysis. However, as models become more integrated and automated in production environments, continuous monitoring and measurement are often overlooked.
Commonly, teams concentrate on performance metrics such as AUC and RMSE, while neglecting other vital factors like the timeliness of training data, model reproducibility, and automated retraining. This lack of comprehensive quality assessment leads to limited visibility for ML engineers and data scientists regarding the various quality dimensions at different stages of a model’s lifecycle. Moreover, this gap hinders organizational leaders from making fully informed decisions regarding the quality and impact of ML projects.
To bridge this gap, we propose defining distinct dimensions for each phase of a model’s lifecycle, encompassing prototyping, training, deployment, and prediction (See Figure 1). By integrating the Service Level Agreement (SLA) concept, we aim to establish a standard for measuring and ensuring ML model quality. Additionally, we are developing a unified system to track and visualize the compliance and quality of models, thereby providing a clearer and more comprehensive view of ML initiatives across the organization. Note that Model Excellence Scores (MES) cover certain technical aspects that are integral to Uber’s overall ML governance.
Model Excellence Scores (MES)
The development and maintenance of a production-ready ML system are intricate, involving numerous stages in the model lifecycle and a complex supporting infrastructure. Typically, an ML model undergoes phases like feature engineering, training, evaluation, and serving. The infrastructure to sustain this includes data pipelines, feature stores, model registries, distributed training frameworks, model deployment, prediction services, and more.
To offer a comprehensive evaluation of model quality across these phases, we created and introduced the Model Excellence Scores (MES) framework. MES is designed to measure, monitor, and enforce quality across each stage of the ML lifecycle. This framework aligns with principles and terminologies common among site reliability engineers (SREs) and DevOps professionals, particularly those used in managing microservices reliability in production environments.
MES revolves around three fundamental concepts related to Service Level Objectives (SLOs): indicators, objectives, and agreements. Indicators are precise quantitative measures reflecting some aspect of an ML system’s quality. Objectives set target ranges for these indicators, and agreements combine all indicators at an ML use case level, dictating the overall PASS/FAIL status based on the indicator results.
Each indicator in MES is clearly defined and has a set target range for its metric value, with a specified frequency for value updates. If an indicator falls short of its objective within a given time frame, it’s marked as failing. Agreements, which encapsulate these indicators, represent the commitment level of the service and provide insights into its performance. Figure 2 illustrates the interconnections between agreements, indicators, and objectives, and how they relate to specific use cases and models.
Different indicators might necessitate varied timeframes for resolution and distinct mitigation strategies. Some may require immediate attention with higher priority handling, especially when performance benchmarks are not met.
It’s also important to note that the roles and responsibilities associated with modeling can vary significantly between organizations. In some cases, a single team may handle the entire process, while in others, responsibilities may be distributed across multiple teams or departments.
At Uber, the responsibility for each model is assigned to a designated primary team. This team receives alerts for any discrepancies or issues related to their model, as outlined in the agreement. Teams have the flexibility to tailor these alerts based on the significance and urgency of their ML use cases. It’s important to note that the quality of one model can influence another, either directly or indirectly. For instance, the output from one model might serve as input for another or trigger further model evaluations. To address this interconnectedness, we’ve implemented a notification system that informs both service and model owners of any quality violations in related ML models.
The interaction between the Model Excellence Scores (MES) framework and other ML systems at Uber is depicted in Figure 3. The MES framework, with its indicators, objectives, and agreements, is built on several key principles:
- Automated Measurability: Every indicator in MES is designed with metrics that can be quantified and automated, ensuring robust infrastructure for instrumentation.
- Actionability: Indicators are not just measurable but also actionable. This means that there are clear steps that users or the platform can take to improve these metrics over time in relation to their set objectives.
- Aggregatability: The metrics for each indicator are capable of being aggregated. This is crucial for effective reporting and monitoring, allowing for a cohesive roll-up of metrics in line with the organization’s Objectives and Key Results (OKRs) and Key Performance Indicators (KPIs).
- Reproducibility: Metrics for each indicator are idempotent, meaning their measurements remain consistent when backfilled.
- Accountability: Clear ownership is attached to each agreement. The designated owner is responsible for defining the objectives and ensuring these objectives are achieved.
We focus on some indicators that haven’t been extensively covered in related literature in Table 1. MES is capable of measuring aspects like fairness and privacy, these topics are out of scope of this discussion. We’ve outlined in the table below how each indicator adheres to these design principles, providing examples of measurable metrics, actionable steps for improvement, and the normalization schemes applied to ensure that the metrics are aggregatable and consistent across different use cases. These metrics are either normalized to a [0,1] scale, converted to a percentage, or maintained on a consistent scale across various applications.
Indicators | Description | Possible Actions | Metric Normalization |
---|---|---|---|
Data Quality | Measures the quality of the input datasets used to train the model. This is a compost score for: – Feature null – Cross-region consistency – Missing Partiitions – Duplicates | – Backfill the missing partitions – Sync the data partitions across different regions and instances – De-duplicate the rows in the data | Each component in the composite score is normalized to the percentage scale |
Dataset Freshness | Measures the freshness of the input datasets used to train the model | – Retrain with fresh input datasets – Backfill input datasets if updated data is available | Scale-consistent |
Feature and Concept Drift | Shift in the target and covariate distribution as well as the relationship between the two over time for a model in production | – Apply weighted training or retrain the model with fresh data – Validate the correctness of upstream feature ETL pipelines | Normalized to [0,1] by using normalized distance metric and importance weights |
Model Interpretability | Measures the presence and confidence of robust feature explanations for each prediction generated by the model | – Enable explanations | Normalized to [0,1] |
Prediciton Accuracy | Prediction accuracy of the model on production traffic (e.g., AUC, normalized RMSE) | – Update training datasets to account for train-serve skew – Check for feature or concept drift | Normalized to [0,1] by normalizing the accuracy metric |
Results
The implementation of the MES framework at Uber has markedly enhanced the visibility of ML quality within the organization. This increased transparency has been instrumental in fostering a culture that prioritizes quality, subsequently impacting both business decisions and engineering strategies. Over time, we have observed substantial progress in adherence to SLAs across various dimensions. Notably, there has been a remarkable 60% improvement in the overall prediction performance of our models.
Moreover, the insights gleaned from the MES metrics have been pivotal in identifying areas for platform enhancements. A key development arising from these insights was the introduction of advanced platform tooling for hyperparameter tuning. This innovation enables the automatic periodic retuning of all models, streamlining the optimization process and ensuring consistent model performance. Such improvements underscore the tangible benefits of the MES framework in driving both operational efficiency and technological advancement
Lessons Learned
In our journey of implementing and monitoring key indicators across all ML teams at Uber, we’ve gleaned several critical insights.
Motivating ML Practitioners: The established framework allowed for a tangible measurement of the impact and efforts directed toward quality improvements. By adopting a standard and transparent reporting system, we created an environment where ML practitioners were motivated to enhance quality, knowing that their efforts were visible and recognized across the organization.
Alignment and Executive Support: Initially, quality measures could be perceived as an additional burden unless they are seamlessly integrated into everyday practices from the outset. Implementing a quality tracking framework sheds light on existing gaps, necessitating extra efforts in education and awareness to address these issues. Aligning with executive leadership was crucial, enabling teams to prioritize quality-focused tasks. This alignment gradually led to a shift towards a more proactive, quality-centric culture across the board.
Balancing Standardization with Customization: In designing the framework, we aimed for a level of standardization that would allow for consistent tracking and informed decision-making over time. However, given Uber’s diverse ML applications, it was also vital to permit customization for specific indicators to accurately reflect the nuances of each use case. For instance, in ETA prediction models, we adopted mean-average-error as a more contextual metric than RMSE. The framework accommodated such customizations while maintaining a standardized approach to reporting for consistency.
Prioritizing Incremental Improvements: Managing the framework across a wide array of use cases posed significant challenges in prioritization. We developed a straightforward prioritization matrix to identify which areas needed immediate attention. Recognizing that a handful of models contribute most to the impact, our focus was on enhancing quality in high-impact use cases first.
The Role of Automation: Maintaining ML quality is resource-intensive, and manually managing models in production can divert efforts from innovation. Automating the production lifecycle, including retraining, revalidating, and redeploying models with fresh data, proved invaluable. This automation not only enhanced model freshness (as indicated by the reduced average age of models), but also allowed teams to focus more on innovation and less on maintenance.
Conclusion
We have developed a comprehensive framework that outlines the key dimensions of high-quality machine learning (ML) models across different stages of their lifecycle. This framework is inspired by Service Level Agreement (SLA) principles and is designed to monitor and ensure the quality of ML models. Importantly, it’s structured to accommodate additional quality dimensions, adapting to emerging use cases and evolving best practices in the field.
Our discussion also encompassed the application of this framework in generating insightful quality reports at various levels of the organization. These reports are regularly reviewed, fostering accountability and offering valuable insights for strategic planning. Crucially, by embedding ML quality within the overall service quality of the associated software systems, we’ve facilitated a shared responsibility model. Applied scientists, ML engineers, and system engineers now collectively own ML quality. This collaborative approach has significantly bridged the gap between these functions, fostering a proactive, quality-focused culture within the organization.
Acknowledgments
We could not have accomplished the technical work outlined in this article without the help of our team of engineers and applied scientists at Uber. We would also like to extend our gratitude to the various Technical Program Managers – Gaurav Khillon, Nayan Jain, and Ian Kelley – for their pivotal role in promoting the adoption and compliance of the MES framework across different organizations at Uber.
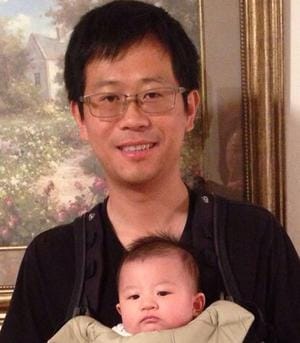
Min Cai
Min Cai is a Distinguished Engineer at Uber working on the AI/ML platform, Michelangelo. He received his Ph.D. degree in Computer Science from Univ. of Southern California. He has published over 20 journal and conference papers and holds 6 US patents.
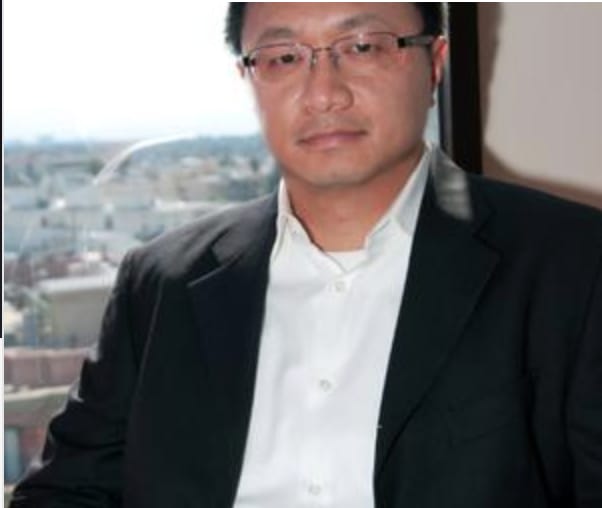
Joseph Wang
Joseph Wang serves as a Principal Software Engineer on the AI Platform team at Uber, based in San Francisco. His notable achievements encompass designing the Feature Store, expanding the capacity of the real-time prediction service, developing a robust model platform, and improving the performance of key models. Presently, Wang is focusing his expertise on advancing the domain of generative AI.
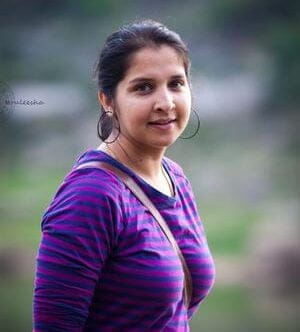
Anupriya Mouleesha
Anupriya has been a thought partner and executor for multiple engineering initiatives within Uber spanning software networking, Data, ML platform, UberAI and product development. She currently leads Technical Program Management for Uber's Mobility and Product Platforms.
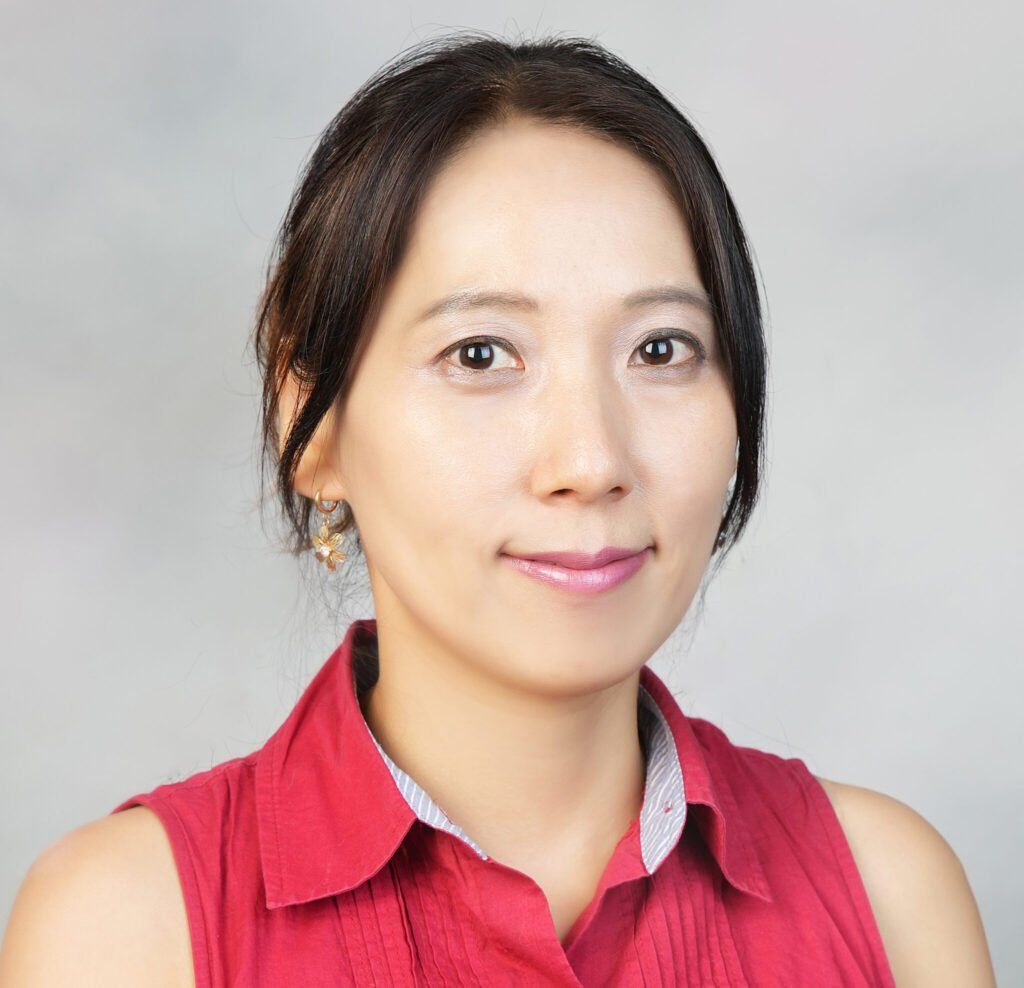
Sally Mihyoung Lee
Sally is a Senior Staff Tech Lead Manager who leads ML Quality and ML Ops for the Uber AI/ML platform, Michelangelo. With over 15 years of experience, she is passionate about applying machine learning solutions to large scale business problems.
Posted by Min Cai, Joseph Wang, Anupriya Mouleesha, Sally Mihyoung Lee
Related articles
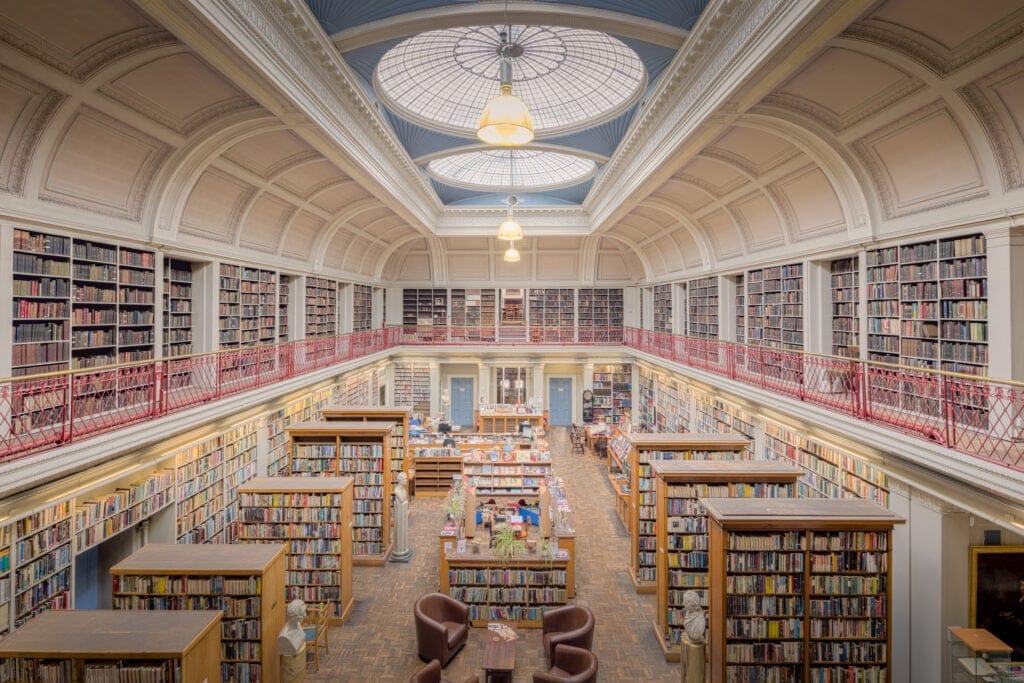
How LedgerStore Supports Trillions of Indexes at Uber
4 April / Global
Most popular
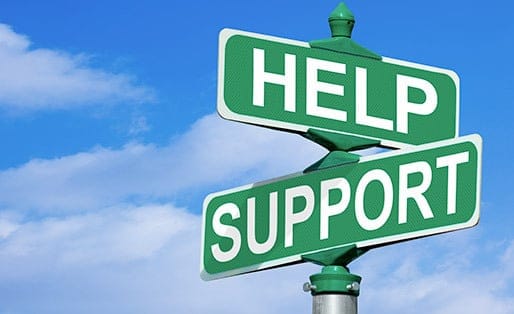
Building Scalable, Real-Time Chat to Improve Customer Experience
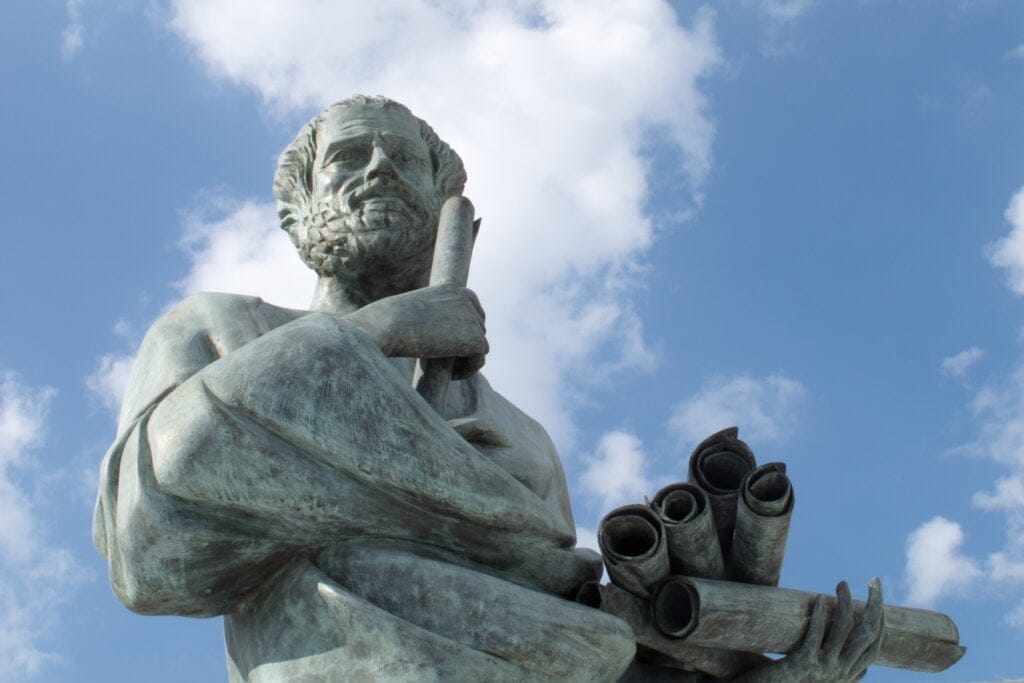
Network IDS Ruleset Management with Aristotle v2
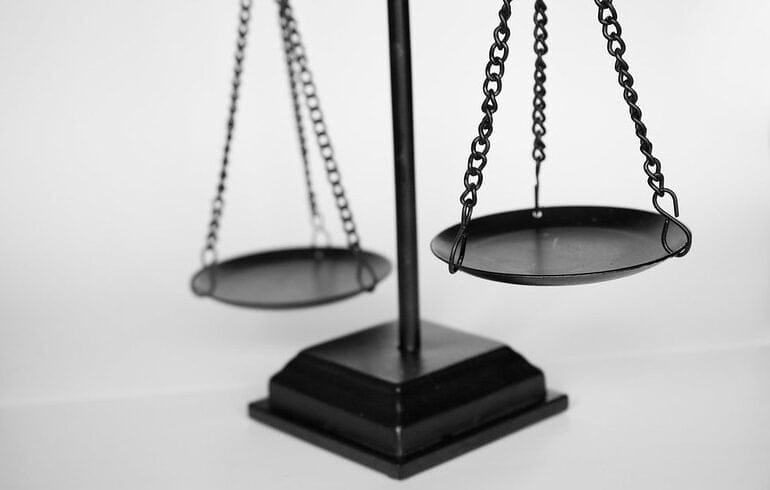
Load Balancing: Handling Heterogeneous Hardware
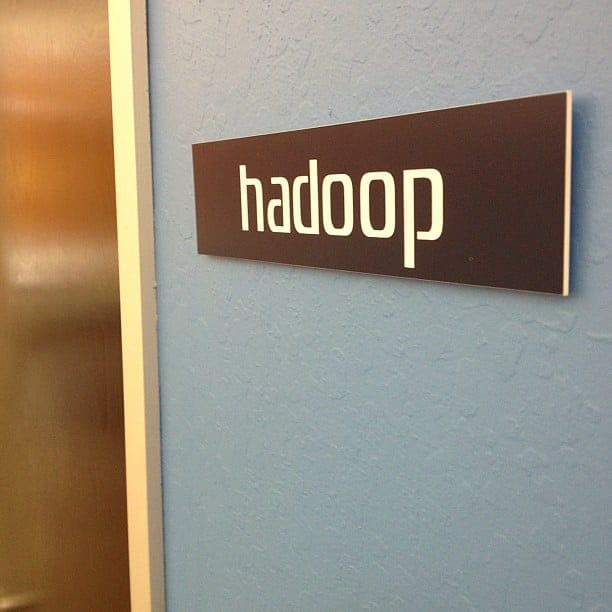
Balancing HDFS DataNodes in the Uber DataLake
Products
Company